This machine learning method can help develop advanced materials for applications such as energy storage and photovoltaics with its highly efficient structure predicting ability.
To develop any product, the properties of the material is required to be understood. The atomic structure plays an important role in understanding the behavior of a material. Based on these properties the products are designed.
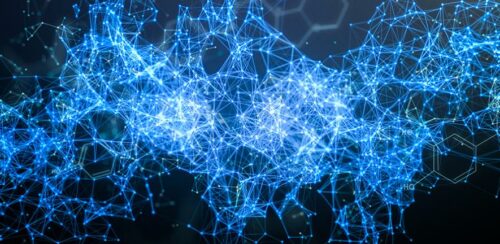
The researchers from the Cambridge and Linkoping Universities, have designed a way to predict the structure of materials based on what elements it is made of. The ability to computationally predict the arrangement of different combinations of elements, without developing any physical model, can help researchers to design materials at a faster and more efficient rate.
However there are many ways that atoms can pack into a material. “There are many possible structures that a material can ‘fold’ into. Except the materials science problem is perhaps even more challenging than biology because it considers a much broader set of elements.” said Dr. Alpha Lee from Cambridge’s Cavendish Laboratory, who co-led the research, relating this problem to the protein-fold problem in biology.
Lee and his colleagues have developed a new way using machine learning to describe materials. That is using mathematics of symmetry to reduce the infinite ways into which an atom can pack into the material.
“The number of materials that are possible is four to five orders of magnitude larger than the total number of materials that we have made since antiquity,” said co-first author Dr. Rhys Goodall, also from the Cavendish Laboratory. “Our approach provides an efficient computational approach that can ‘mine’ new stable materials that have never been made before. These hypothetical materials can then be computationally screened for their functional properties.”
This research can help find some new materials that can be more stable and have never been used before. This paves the road to better technology for energy storage and photovoltaics as well.
Reference : https://www.science.org/doi/10.1126/sciadv.abn4117