Researchers have developed a way to fabricate ECRAM at a low cost for practical AI accelerator applications.
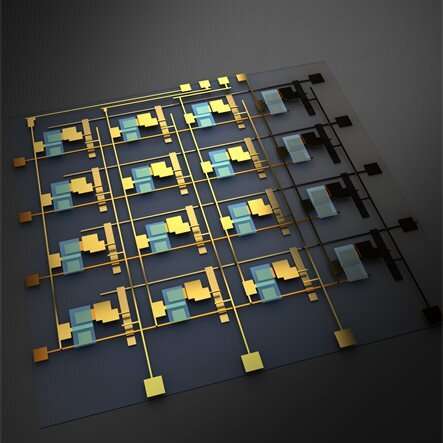
Although Chat-GPT may have brought ease and transformation to our work and the system, it has a very high cost to answer your questions. ChatGPT algorithm costs at least $100,000 every day to operate. This could be reduced with accelerators, or computer hardware designed to efficiently perform the specific operations of deep learning. However, such a device is only viable if it can be integrated with mainstream silicon-based computing hardware on the material level.
A team of researchers at University of Illinois Urbana-Champaign have achieved the first material-level integration of electrochemical random-access memory, ECRAMs onto silicon transistors. ECRAM is a memory cell, or a device that stores data and uses it for calculations in the same physical location. This non-standard computing architecture eliminates the energy cost of shuttling data between the memory and the processor, allowing data-intensive operations to be performed very efficiently.
ECRAM encodes information by shuffling mobile ions between a gate and a channel. Electrical pulses applied to a gate terminal either inject ions into or draw ions from a channel, and the resulting change in the channel’s electrical conductivity stores information. It is then read by measuring the electric current that flows across the channel. An electrolyte between the gate and the channel prevents unwanted ion flow, allowing ECRAM to retain data as a non-volatile memory.
Researchers selected materials compatible with silicon microfabrication techniques: tungsten oxide for the gate and channel, zirconium oxide for the electrolyte, and protons as the mobile ions. This allowed the devices to be integrated onto and controlled by standard microelectronics.
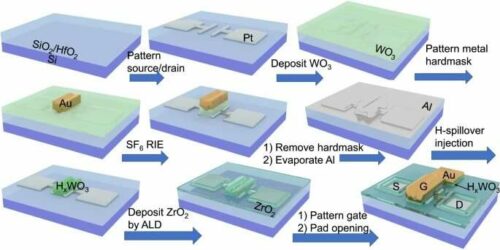
Since the same material was used for the gate and channel terminals, injecting ions into and drawing ions from the channel are symmetric operations, simplifying the control scheme and significantly enhancing reliability. The channel reliably held ions for hours at time, which is sufficient for training most deep neural networks. Using protons enables rapid switching. Researchers found that their devices lasted for over 100 million read-write cycles and were more efficient than standard memory technology. Finally, since the materials are compatible with microfabrication techniques, the devices could be shrunk to the micro- and nanoscales, allowing for high density and computing power.
Researchers demonstrated their device by fabricating arrays of ECRAMs on silicon microchips to perform matrix-vector multiplication. Matrix entries (neural network weights) were stored in the ECRAMs, and the array performed the multiplication on the vector inputs, represented as applied voltages, by using the stored weights to change the resulting currents. This operation as well as the weight update was performed with a high level of parallelism.
Reference : Jinsong Cui et al, CMOS-compatible electrochemical synaptic transistor arrays for deep learning accelerators, Nature Electronics (2023). DOI: 10.1038/s41928-023-00939-7