Researchers at the University of California have developed a system that trains small robotic cars to drive autonomously at high speeds.
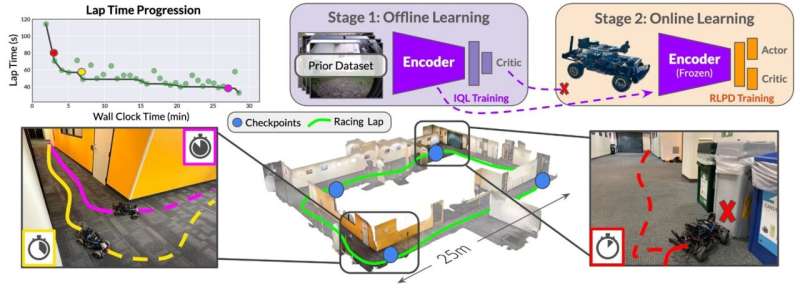
Fast cars have been adored for over a century and unite enthusiasts regardless of nationality, race, religion, or politics. From the classic Stutz Bearcat and Mercer Raceabout in the early 1900s to the iconic Pontiac GTOs and Ford Mustangs of the 1960s to today’s luxurious Lamborghini and Ferrari vehicles. Robotic cars are now joining the excitement.
Researchers at the University of California at Berkeley have developed an inaugural system instructing small robotic cars to self-drive at high velocities and enhance their skills in real-world settings. Fast reinforcement learning via autonomous practicing (FastRLAP) trains independently in the real world without human intervention, simulation, or expert demonstrations.
First, an initialization stage gathers data on various driving environments. A manually operated model car navigates through diverse courses prioritizing collision avoidance, not speed. The car that learns to drive fast does not have to be the same. A robotic car is sent on a course it needs to learn after a dataset covering a wide range of routes is collected. It begins with a preliminary lap to establish a perimeter before proceeding independently. Using the dataset, reinforcement learning (RL) algorithms train the car to avoid obstacles, improve efficiency, and adjust speed and direction.
According to the researchers, robotic cars could master racing courses in less than 20 minutes of training. The team noted that the results display “aggressive driving skills,” like timed braking, accelerating on turns, and avoiding obstructions. The robotic car’s skills match human drivers who use a similar first-person interface during training. The researchers explained that the robotic car finds a smooth path through the lap, increasing its speed in tight corners. It learns to decelerate before turning, then accelerates out of the corner to reduce driving time. In another example, the car learns to slightly oversteer on low-friction surfaces, “drifting into the corner” to rotate quickly without braking during the turn.
To improve RL-based systems’ ability to learn complex navigation skills across domains, the researchers suggested addressing safety concerns. The current system only prioritizes collision avoidance to prevent task failure in familiar environments.
Reference : Kyle Stachowicz et al, FastRLAP: A System for Learning High-Speed Driving via Deep RL and Autonomous Practicing, arXiv (2023). DOI: 10.48550/arxiv.2304.09831