Researchers from Oxford, IBM Research Europe, and the University of Texas have developed thin artificial neurons for light and electric signal computing.
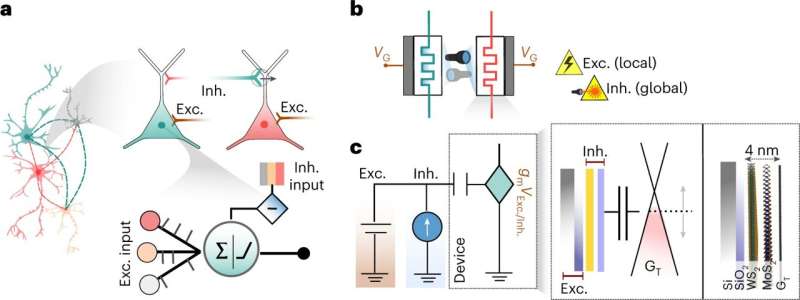
Memristors, electronic components that store values by modifying their conductance, are a promising solution for in-memory processing. Replicating complex cognitive processes with memristors has been challenging because integrating feedforward and feedback signals is essential for learning complex tasks involving rewards and errors.
Researchers at the University of Oxford, International Business Machines (IBM) Research Europe, and the University of Texas have created atomically thin artificial neurons that can process light and electric signals for computing. This material allows for separate feedforward and feedback paths in a neural network, enhancing the capacity to solve intricate problems. The researchers have made memristors responsive to optical and electrical signals, expanding their functionality and enabling separate feedforward and feedback paths. This breakthrough resulted in winner-take-all neural networks capable of solving complex machine learning problems, including unsupervised learning in clustering and combinatorial optimization problems.
By layering just a few atoms, 2D materials exhibit exotic properties that can be fine-tuned. Researchers used graphene, molybdenum disulfide, and tungsten disulfide to create a device that changes conductance based on the duration and power of light/electricity. Unlike digital storage devices, these analog devices mimic biological synapses and neurons. They compute through gradual changes in stored electronic charge caused by a sequence of electrical/optical signals. The researchers believe that the study introduces a novel concept exceeding current artificial neural networks’ fixed feedforward operation with promising applications in AI hardware. These results also mark a significant scientific advancement in neuromorphic engineering and algorithms, enhancing our understanding and emulation of the brain.
The researchers created atomically abrupt semiconductor junctions using their heterostructure stack, where the heterojunction acts as the neuronal membrane and graphene electrodes as the neuronal soma. This design represents the soma’s neuronal state, modified by membrane changes, mimicking actual neurons. This exciting field combines material and device innovations with creative applications, requiring the convergence of these elements. The new toolkit presented in this work explores the potential of 2D materials in novel computing paradigms rather than transistors.
The researchers believe that their findings are exploratory rather than demonstrations at the system level. While they plan to expand on the concept in the future, their proof-of-principle results are significant for neuromorphic engineering, enhancing comprehension and emulation of the brain.
Reference: Ghazi Sarwat Syed et al, Atomically thin optomemristive feedback neurons, Nature Nanotechnology (2023). DOI: 10.1038/s41565-023-01391-6