- High-parameter AI models excel in accuracy but worsen energy inefficiency in conventional processors.
- A chip with 35M memory devices and 34 tiles attains 12.4 TOPS/W (tera-operations per second per watt ), showcasing software-equivalent accuracy for keyword spotting and near-equivalent accuracy for large neural networks across five chips.
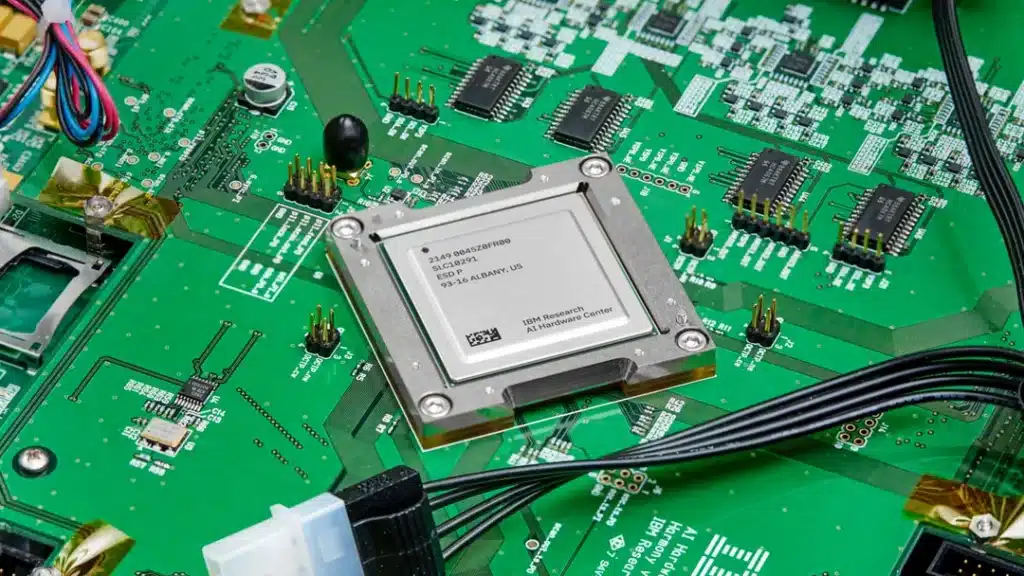
IBM has unveiled an artificial intelligence (AI) advancement by developing analog AI chips that exhibit energy efficiency, potentially revolutionizing AI technology. These chips have demonstrated an estimated 14 times greater energy efficiency than conventional digital AI chips, offering promising prospects for reducing AI’s carbon footprint. AI technology has already initiated a transformative shift in various aspects of lives; however, it is accompanied by a significant energy demand. Some estimates indicate that running large AI models can produce more emissions over their lifetimes than the average American car. Addressing this energy consumption is crucial to align AI progress with global climate goals.Â
The Birth of Analog AI Chips
The team has pioneered this approach by utilizing analog chips, which consume substantially less power. They introduced their prototype analog AI chip for energy-efficient speech recognition and transcription. In two AI inference experiments, these analog chips matched the performance of their digital counterparts and completed tasks more quickly while consuming less energy. While the concept of analog AI chips for inference isn’t new, previous iterations did not address the needs of today’s massive AI models, such as GPT-3, which can have up to 175 billion parameters. This design can accommodate 35 million phase-change memory devices per chip, equivalent to models with up to 17 million parameters. By optimizing multiply-accumulate (MAC) operations through resistive non-volatile memory (NVM) devices, these analog chips perform MACs within the memory, eliminating the need to transfer weights between memory and compute regions. This parallel operation not only saves time but also conserves energy.
Two Key Experiments
The team conducted two experiments to evaluate their analog AI chip’s performance. The first experiment focused on keyword utterance detection, showcasing the chip’s ability to recognize specific spoken words accurately and quickly. The second, more substantial experiment utilized five chips to transcribe spoken language, with results suggesting that analog AI could potentially replace digital systems for complex tasks while maintaining high energy efficiency. Comparing their prototype to digital hardware using data from MLCommons, they found that their analog AI chip outperformed digital systems by a significant margin, with an estimated 14 times higher performance per watt.The potential applications of analog AI extend beyond natural language tasks. They demonstrated the chip’s computer vision image recognition capability, emphasizing its versatility.Â
Although this development marks a substantial stride toward energy-efficient analog AI systems, there is ongoing work to integrate the two workstreams and adapt foundation models to these chips. As analog AI continues to evolve, it promises to address AI challenges while minimizing its environmental impact, offering a vision of a future where power-conscious analog AI coexists with existing digital systems.