Over the past few decades, innovative memory architectures have shaped memory design for various physical devices. This change continues and is particularly interesting from the perspective of artificial intelligence, The Internet of Things, and robotics.
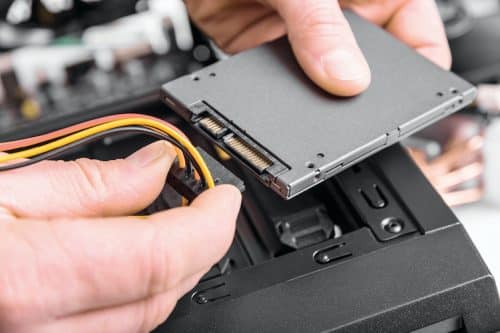
System development has come a long way over the years. The process began right when computers were being designed. In a system, the critical components are the processor and memory. When both of these are efficient, you get more processing power and better bandwidth for memory. Already a lot has happened on the processing side with the central processing units (CPUs) evolving into graphics processing units (GPUs) and later transitioning into neural processing units (NPUs).
This has come into effect in mobile phones with the introduction of NPUs for faster artificial intelligence (AI) processing. It seems the maximum limit has been reached there. Now the focus is shifting towards strengthening the memory efficiency. This is where 3D SRAMs and stack DSRAMs are coming in. “I will not be surprised if intelligent memory starts coming in the future. We have not reached that point yet, but we have come to a stage where we are thinking of pipelined design in software,” says Nikhil Bhaskaran, founder, Shunyaos.
Neural networks
Neural networks certainly hold promise in developing intelligent memory for faster data compute and efficiency. Huge datasets require frequent memory access. Doing that in today’s memory system creates high inefficiency, leading to speed loss. To overcome that, AI on edge offers a viable solution where a fast output can be obtained. “This correlates with testing the limits of a system, but that is when things start getting fixed,” says Nikhil.
The future is about AI happening completely on the edge where both the processor and the memory need to be efficient. “They are like husband and wife. One cannot go too fast, otherwise the marriage will break. Everything has to happen in tandem,” explains Nikhil.
Convolutional neural networks (CNNs) do a lot of processing via multiply-accumulate (MAC) operations (which is to multiply two values and store the result in the accumulator). Here the value has to be held for a certain time while the next operation is being performed. But if the time taken to deliver and receive that value is more than its processing speed, then there is a problem. One solution is to bring memory closer to a system, so that with the interface pipelines the bandwidth speed can be increased. This is highly required as we increasingly move towards edge computing.
Memory from the IoT perspective
IoT generally involves communicating, processing, and storing data. And although microcontrollers can effectively handle embedded systems, they cannot handle high-speed data control, transfer, and storage required for communication with the cloud. Therefore, memory architecture needs to be strengthened for IoT. “An embedded system is required that has low limitations with respect to size, data as well as power,” says Dharmendra Kumar, IoT & Digital Business Head at Arihant.
He strongly recommends design engineers to go for such memories as EMC and universal flash storage (UFS), which have both microcontroller and intelligence inside them. “Memory from an IoT point of view requires the presence of intelligence not just for the software but also for the hardware,” he emphasises.
In recent years, mobile phones have emerged as powerful tools for enabling seamless functioning of IoT devices. So, if a simple operation such as switching on/off an IoT device is required, the related data can then be stored on the device itself without memory. But if a complex process such as data intelligence and data acquisition from the sensor needs to be carried out, then memory is required.
At the same time, some cases need network considerations as well. Take for example narrowband IoT (NB-IoT). It is a part of the GSM technology in which data is in a constant state of motion. So why not eliminate the need for external memories and simply place the memory within the NB-IoT module? For that to happen, the GSM platform as well as the embedded system can be interfaced via the concept of host microcontroller. How much data is suitable for traveling from hardware to software in an embedded system also requires careful consideration.
Memory for mobile robotics
The efficiency of a robot determines the quality of the work output, for which there should be intelligent processing and controlling. To achieve this, memory management and organisation is important. A communication interface between the operating system and the embedded software is needed for effective synchronisation between two or more different platforms.
In an industry, typically there are multiple workstations, each having different needs. For example, one workstation calls for materials from stores, another for transportation of finished goods to a warehouse, and so on. Here, the industrial mobile robot employed to carry out such tasks should be able to do so effectively from one workstation to another.
Since it is difficult to predict the nature and application of the calls in such a scenario, these should be prioritised based on risk and urgency, which requires intelligence in the robot. If the task is of high risk or high urgency, then it should be given the first priority.
This assigning of priorities is similar to weighing of the neural network systems. Weights are assigned here in the form of call requests. Other than that, single-board computers can be employed for queue mechanism for which a stack can be present to perform the memory organisation and call request management. There should also be a mobile robot localisation part to implement locomotion for the robot movement.
“Localisation means that there should be an obstructionless path for the robot to move. Robot condition is also important to keep in check the wear and tear mechanism and alignment problems. Everything is monitored using inertial measurement and position sensors. From these sensors we can implement a sensor-fusion algorithm. Based on this algorithm, prioritisation of call management and memory organisation helps improve the intelligence and efficiency of the robot, which can also be considered as an intelligent smart mobile robot with trolley-type material transportation system,” says Vishnu Kumar, Freelance Consultant at Embedded Systems and Mobile Robotics.
All these things determine how a robot can autonomously work in a certain environment.
As processing speed requirements are increasing day-by-day, it is critical for accessing the memory from the processor and other peripherals. At the same time, for efficient power management and consumption, certain algorithms should be improvised. Normal battery power is enough for today’s robotic needs, but in future a number of motors and multiple power handling devices will be required. Hence, thought should also be given to power management. The size and weight of the power device is also quite important.
Edge device design and manufacture
The government of India is taking a lot of initiatives to promote manufacturing under its Make in India programme. But the challenge while focusing on embedded systems or modules is that there is insufficient support for memory production and manufacturing ecosystems in India. These need to be developed.
Dharmendra informs that in some other countries, research involving nanotube RAM (NRAM) is going on. So, India should also go for NRAM and adopt this technology.
Technologies such as ReRAM and NRAM have been developed, but India is lagging behind with respect to these. As the development platform exists in the country, it should be utilised. “Although we are making certain efforts, we need the ecosystem. Government is taking a lot of initiatives, but they need to support the ecosystem even for design,” he says.
On a similar note, Nikhil feels that emphasis should be given more on design than on manufacture. Just as China is good at manufacturing, India is good at designing. “If we have to be a leader, then we have to mass educate on the latest technologies rather than holding them. That is all what is required for India to become a leader in design,” he says. He thinks that to fulfil our manufacturing aspirations, we should take inspiration from the Chinese manufacturing industry while staying practical with respect to our strength, which is design.
Further insights
The next level of innovation is going to be in quantum computing. Since quantum is multi-dimensional, an intelligent chip is needed that will be able to do all the management.
On the possibility of increasing the RAM capacity in a given device, the emergence of ReRAM can help increase it, especially for space-constrained areas and where the data rate is remarkably high.
In conclusion, AI on edge plays a vital role in enhancing the memory design for developing robust embedded systems. In the sphere of IoT, a strong data communication equipped with a reliable data storage and privacy mechanism should be there. For mobile robotics, a well-defined memory organisation determines the intelligence of a robotic system to prioritise a task. With these in place, the time to come is surely going to be really exciting as it will bring with itself new opportunities for the next generation of the advanced driver-assistance systems, artificial intelligence, and memoryless ecosystems.
This article is based on the panel discussion ‘Memories: What’s New for Design Engineers?’ in November edition of India Technology Week 2020. The panel was chaired by Naresh Neelkantan, senior architect – system software at Sasken Technologies