The novel framework achieves state-of-the-art performance without sacrificing efficiency in public surveillance tasks
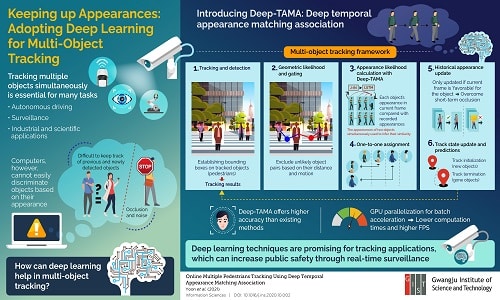
Computer vision has progressed much over the past decade. Implementing algorithms for simultaneous tracking of multiple objects is essential for applications involving autonomous driving and advanced public surveillance. However, computers often find difficulty in accurately distinguishing between detected objects. One example is object tracking, which involves recognising persistent objects in video footage and tracking their movements. While computers can simultaneously track more objects than humans, they usually fail to discriminate the appearance of different objects, leading to algorithm mix up of objects in a scene and producing incorrect tracking results.
Now, researchers at the Gwangju Institute of Science and Technology (GIST) have adapted deep learning techniques in a multi-object tracking framework, overcoming short-term occlusion and achieving remarkable performance without sacrificing computational speed. The new tracking model is based on a technique called ‘deep temporal appearance matching association (Deep-TAMA)’, which promises innovative solutions to some of the most prevalent problems in multi-object tracking.
Conventional tracking approaches determine object trajectories by associating a bounding box to each detected object and establishing geometric constraints. The inherent difficulty in this approach is in accurately matching previously tracked objects with objects detected in the current frame. Differentiating detected objects based on features such as colour usually fails because of changes in lighting conditions and occlusions. Thus, the researchers strengthened the tracking model to accurately extract the known features of detected objects and compare them not only with those of other objects in the frame but also with a recorded history of known features. To this end, they combined joint-inference neural networks (JI-Nets) with long-short-term-memory networks (LSTMs).
LSTMs helps to associate stored appearances with those in the current frame whereas JI-Nets allow for comparing the appearances of two detected objects simultaneously from scratch, allowing the algorithm to overcome short-term occlusions of the tracked objects. “Compared to conventional methods that pre-extract features from each object independently, the proposed joint-inference method exhibited better accuracy in public surveillance tasks, namely pedestrian tracking,” said Dr Moongu Jeon, professor at the School of Electrical Engineering and Computer Science, Gwangju Institute of Science and Technology (GIST).
Moreover, tests on public surveillance datasets confirmed that the proposed tracking framework offers state-of-the-art accuracy and is therefore ready for deployment.
Multi-object tracking unlocks a plethora of applications ranging from autonomous driving to public surveillance, which can help combat crime and reduce the frequency of accidents. “We believe our methods can inspire other researchers to develop novel deep-learning-based approaches to ultimately improve public safety,” concludes Dr Jeon.
For more, visit here.