Open computing language, or OpenCV, as the name suggests, is an open source software that aims at computing efficiency and bettering real-time computer vision. An image processing software, OpenCV, helps us achieve whatever we want to do with images, right from understanding these to completely ripping these apart.
What can you do with OpenCV
Images can be of two kinds, namely, hand drawn or captured. In both cases, when we are dealing with images, there are two things we might want to do: understand the images completely and make these visually appealing.
OpenCV lets you do both, by providing a variety of functions and features, which, when used correctly, give the desired result. With more than 2500 optimised library algorithms and new algorithms that can be written easily, it lets you work with images in an easy and comfortable manner.
Masking. A mask is a spatial filter. When we need to hide a certain region of an image, we can use a corresponding mask. It is like using a black-and-white filter on the image, wherein the black region blocks out the image, while the white region acts as a transparent sheet. An example can be seen in Fig 1.
Contrast and brightness. To enchance the image’s appeal, we can play around with brightness and contrast features. Changing the contrast changes the difference between the selected region and its background, while brightness changes the appearance. We use the two to please our visual perception.
Smoothing, blurring, blending and sharpening. The anti-aliasing approach that finds usage right from image to audio processing is similar to smoothing and blurring the image.
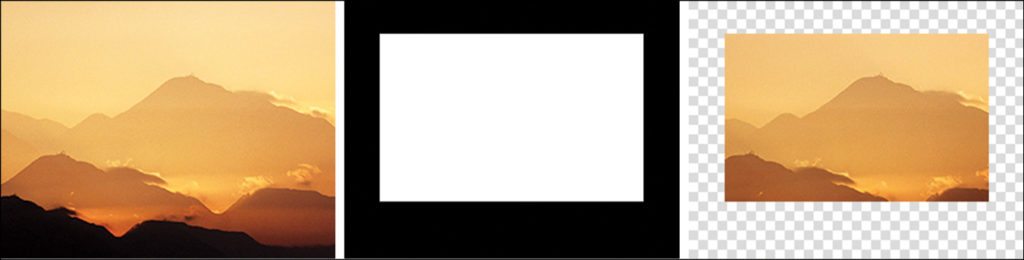
Smoothing and blurring help us nullify the difference between jagged edges and the rest of the image. These aid in generating background effects, shadows and even achieve region highlighting.
Blending involves mixing two layers by placing one on top of the other. Depending on the opacity of the two layers, the resulting effect can be hiding the bottom layer or dissolving the two or a transparent top layer. The reverse process; if we want to recover fine details of an image that is blur or low on resolution, we can sharpen the image.
Add noise. OpenCV lets us add a realistic angle to the image by corrupting it. This is like simulating the setup, taking into account noise from the detector, salt-and-pepper noise or data-transmission noise and also image-dependent noise.
Boost, identify and recognise. We can follow eye movements in a video by tracking or measuring the point of gaze or the motion of the eye relative to the head. Removing red eyes in an image taken using flash is also a simple task. Boosting image classification by categorising images that can be used as a base in future. We can recognise scenery, as the tool compares the new image with previously-recorded ones.
The same goes with identifying or tagging people. Tracking objects and detecting motion in videos also take place using the same process.
Segmentation, edge detection and morphology. With this tool, the image under processing can be partitioned into multiple segments by using the thresholding approach. Also, points, lines and edges in an image can be easily detected by simply applying different order derivatives to the image. Fig. 2 shows what an edge-detected image looks like.
Morphological operations process the image based on shapes or templates called structuring elements, and we can apply image pyramids to either upsize or downsize the image.
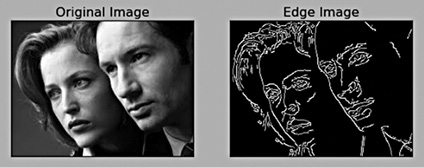
Histogram. Analyse your image by obtaining the image histogram and studying the intensity distribution. The tool scans the entire image, tabulates different intensities and increases the corresponding intensity count as it scans the image, simultaneously. Fig. 3 shows a sample histogram.
We can use this information, which is generally seen as a graph, to fix threshold limits and decide the pixel adjustments to be made to the image. The histogram can also be equalised to improve image contrast.
Transformations. Convert the image from the existing spatial domain to corresponding frequency domain by applying Fourier transform. To find imperfect instances of objects, like circles and other classes of shapes in your image, apply Hough transform. The image can also be remapped by moving existing pixels to a new image.
The complex math and a little beyond. Statistical evaluation using expectation-maximisation algorithm, k-nearest neighbour algorithm, random forest and support vector machine is made easy by simply coding it into the tool and then applying the same on the image.
Decision tree learning can be used to predict an image’s target value by mapping observations. Multi-dimensional scaling, naive bayers classifiers and gradient boosting help you work with the images better. Image analysis can even be extended to the level of neural networks, converting neuron connections to numeric weights that can be worked with.
If you want to make your own videos with existing images, you can interact with and manipulate the real world around using augmented-reality feature.
The added modules. We can create three dimensinal (3D) reality from two dimensional (2D) images using Calib3d. Point detectors, descriptors and matching framework options can be found in Feature2d. Algorithms to perform motion extraction, feature tracking and foreground extractions on the video stream are provided in the video module.
Graphical processing. An exclusive graphical processing unit (GPU) module helps squeeze out every little computation power from the system by using the power of the video card to run OpenCV algorithms. Based on compute unified device architecture (CUDA), the module provides GPU acceleration and helps developers run more accurate and sophisticated OpenCV algorithms in real-time on higher-resolution images, while consuming less power.
Added support to OpenCL in the library enables the codes to take advantage of the heterogeneous hardware, in particular utilise the potential of discrete and integrated GPUs.
Graphical user interface (GUI). The built-in GUI allows easy access to the functionalities of OpenCV. We can add track bars to applications and also read videos or create new ones. There are also methods to perform similarity measurements to check if two videos or images are copies of each other.
Pointers from the latest release
OpenCV 3.0 gold release, as it is popularly called, has a transparent application program interface, which is a GPU-acceleration layer using OpenCL, introduced with support from AMD and Intel. With access to a sub-set of Intel’s integrated performance primitives, improved Python and Java bindings, new MATLAB bindings and accelerated functions using NEON intrinsics, it promises to be the most functional and fastest OpenCV ever.
Projects from Google Summer of Code programme have been integrated into this release. Biologically-inspired vision module, DAISY features, LATCH descriptor, improved BRIEF and image registration module are contributions from communities.
Further, features like text detection, line descriptors, general use optimisation (hill climbing and linear programming), computational photography algorithms (HDR, in-painting, edge-aware filters and superpixels), tracking and optical flow algorithms, 2D shape-matching module and 3D surface-matching module, RGB-D module and visualisation tool-kit based 3D visualisation module have been introduced.
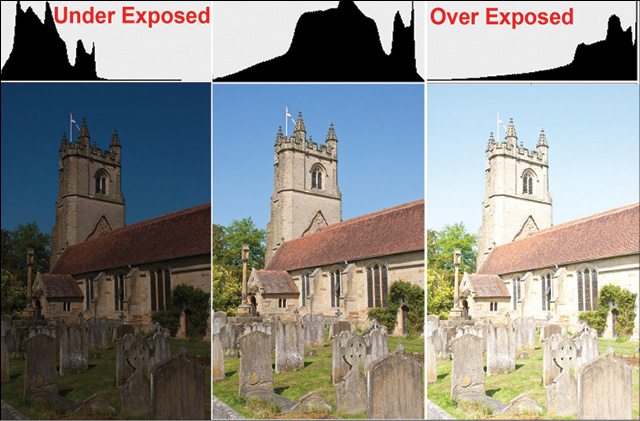
Installation and setup
The installation process of OpenCV involves installing libraries and packages for different platforms. Although it seems complicated, tutorials on the website guide you with step-by-step details of doing the same. The library can be downloaded from an already existing setup or one can even create his or her own library from scratch.
The latest version OpenCV 3.0 can be downloaded from SourceForge, for various platforms, as follows:
Windows self-extracting archive: click here
iOS framework:Â click here
The source code for all platforms can be downloaded from GitHub: Â click here
Various books have been authored exclusively on OpenCV. Getting a hand on one might prove to be just what we need to work our way through this software. There are books like OpenCV for Secret Agents, Computer Vision with OpenCV and Learning OPenCV, to name a few. Books even come in platform-specific versions. One can take a look at the books on opencv.org/books.html
What the users have to say
OpenCV seems to be top-notch when one takes into account first-hand experience. Starting from installation to running the tool, the process turns out to be smooth. Easy-to-read documentation, a powerful and well-designed library and great algorithms appeal to the user.
What works in OpenCV’s favour is the user-friendly software and multiple features, along with regular updates. Good quality, being stable in terms of functionality and fast working pace make people adapt to it easily.
GPU module is a value addition. Some go on to say that, with just a little improvement on the GUI front and specific documentation sections, the tool could even become the future of image processing. No wonder then that OpenCv has a rating of 4.8 on 5 from SourceForge.
The website provides documentation and tutorials for every possible operation that can be performed within OpenCV. An answers page allows you to post questions or replies to others’ questions. Even after repeated attempts, if bugs are not solved, you can report these or request new features to be added, on the website. You can find OpenCV projects at code.opencv.org/projects/opencv
If you want to try out other tools, you could go for VLFeat, WEKA or ToolIP. Try OpenCV to play with your images and make these fit for your application.
Download latest version of the software: click here
Priya Ravindran is a technical journalist at EFY