Recent advancements in state-of-the-art technologies have increased the popularity of intelligent self-driving cars and electric vehicles. Yet their ability to face several demanding factors while on the road is fraught with complications that need to be resolved.
India is famous (or infamous) for having poor road conditions and traffic that can serve as a perfect testing ground for intelligent vehicles anywhere in the world. But the country is quite behind in their acceptance despite possessing experts with outstanding technical knowledge. At the same time, there has been significant move and investments towards smart precincts and smart cities over the recent past.
Meanwhile, electric vehicles (EVs) are gradually witnessing some degree of recognition among the general public thanks to extensive awareness initiatives by the government and large-scale support by various corporations.
Current EV trends
IC engines used to follow different categories of standards. Since shifting to electric vehicles, these standards have changed. Things have now moved on to higher voltage ranges of 72V to 700V from lower voltages like 12V and 24V. With this the required test infrastructure and facilities have entered a new phase, ushering in the era of EV adoption.
“With many more EVs to come on the road, the amount of feedback coming out from the vehicles in real-world scenarios will increase. This data is crucial for improving future system designs and increase the EV adoption,” says Sujit Yardi, chief engineer, Devise Electronics.
AC charging stations in households can easily employ a 15A in-house plug for charging an EV. Several end-point delivery companies such as Amazon, Flipkart, and Swiggy use this model. So rather than a CAPEX model, people are going for an outsourced (or OPEX) model where the vehicle is not purchased but rented. In the coming years, the OPEX model is going to be more popular than the CAPEX model.
At present, consolidation of the standard protocols is taking place on a global scale for the EVs and charging stations so that a single charger can be used anywhere, preventing any confusion caused by the use of different charging standards. As of now Japan uses CHAdeMO while Tesla uses its proprietary connectors. We see CCS2 (combined charging system) being used in Nexon EV and MG ZS EV. Down the line, most of the manufacturers will migrate to CCS2 standard, which is compatible with both AC and DC charging stations/piles.
On the standards that EV makers have to comply with in India, the Automotive Research Association of India (ARAI) has formulated different standards for different vehicle categories (two-wheeler, three-wheeler, four-wheeler, and heavy electric vehicle). These are ‘open’ and freely available, motivating multiple startups to come up with new classes of EVs.
Startups looking to launch their products in the market can take help of gyaniki, a knowledge portal based on past experiences of automotive designers, where a lot of information is given on EVs, autonomous vehicles, and related component manufacturers.
Intelligent vehicle challenges
Recounting his PhD days in the UK back in 2010, Dr Rahul Kala, now an assistant professor at the Centre of Intelligent Robotics – IIIT Allahabad, says that one day while walking from his residence to workplace, he witnessed chaos in a nearby street. Due to some reason, one of the lanes in a two-lane British road was blocked. Yet the drivers cleverly managed to get past each other without crashing. This made him think… can self-driving cars do the same?
Considering this scenario, it can be interpreted that autonomous machines fail to produce the same level of perception at present. Humans generally deal with machines through non-verbal communication, which is unlike a verbal human-to-human conversation. Vehicle intelligence is not based on the number of miles tested but on gauging the level of human intelligence (or ignorance) around testing. And the only way of knowing it is by analysing the behaviour of people with poor driving skills around those with good driving skills. This test can enable an intelligent vehicle to match up to the chaos that exists on roads.
Another thing to note is the uncertainty of local weather conditions. All in all, it is a question of dealing with the unpredictability of humans and nature. If the situations are handled well, then it can safely be said that we have reached the levels of testing in autonomy.
“Observation is the key. The more you observe, the more key points you get for validating the test cases,” says Naresh Neelakantan, senior architect – system software at Sasken Technologies.
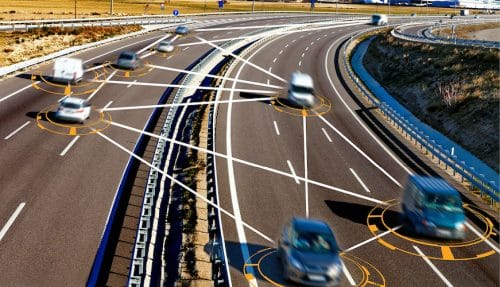
Cloud computing challenges
Autonomous cars together utilise multiple sensor inputs to collect enormous data through vision processing and object detection to prevent road accidents. However, the biggest challenge is efficiently processing and delivering high data volumes on the existing communication network. Even if 5G is available everywhere, it cannot provide the required low-enough latency. A delay of even a millisecond could result in a fatal collision.
“What happens if the data connectivity fails while on the road? We cannot rely on the cloud infrastructure for every process,” says Arqum Ahmad, CEO, Antrax Labs and AI scientist at Leonardo, Italy (Military Aircraft Division). “We need an autonomous car that can perform object detection and relevant decision making without any delay. This challenge is not just limited to handling the ever exploding data but also ensuring security and reliability.” And this is where edge computing plays a huge role. Edge computing offers low latency and faster decision making on the local site. Keeping this in mind, the Automotive Edge Computing Consortium (AECC)—a group of tech giants like Google and Tesla, universities, and start-ups—has been established to focus on convergence between vehicles and the computing ecosystem.
NVIDIA has already launched a product called Drive DGX, which is an edge node having faster GPUs and to which multiple sensors can be connected. One can use it as a testbed and train a model on the autonomous car itself. Edge computing also plays an important role in EVs. With the help of predictive maintenance, anything going wrong with the battery can be determined (overheating is a big issue). And not just prediction, edge computing also facilitates optimisation of the charging process.
This is not to undermine the importance of cloud computing. Mechanisms such as machine-to-machine communication, smart traffic lights, and other smart city infrastructures rely on cloud computing for effective network connection. Naresh from Sasken agrees and adds, “Unless you have nearby communication infrastructure and bandwidth, the realisation becomes overhead. It is similar to a clogged entry and exit to huge apartments or office spaces.”
Securing IoT for test
While there are different concepts about connected cars, it is simply based on four kinds of bi-directional communication with Internet Protocols (IPs): vehicle to users, vehicle to cloud, vehicle to infrastructure, and vehicle to vehicle.
From a validation standpoint, security concerning cloud, edge, and embedded design, and user acceptance with respect to features play a major role. In absence of a testing infrastructure, it would be unfeasible to test driverless cars. And if we test in the lab, then how would the car enter market? Therefore, we need to define the regulation, security levels, and connectivity protocols before moving towards the edge for secure testing.
Sensor ecosystem for wireless connectivity
First, a rugged testing bench for the hardware needs to be created that directly interfaces with the diagnosis system of a car for capturing data (people can use DSP and microcontrollers). Second, for software compatibility of the hardware, firmware-over-the-air (FOTA) needs to be implemented to update data within the hardware itself (while carrying out data and embedded communications).
Coming to wireless connectivity, the best way for validating a connected car is through edge computing. It can be entirely within the car (as a hardware part) or on the cloud for data transfer and acquisition. On the sensing side, smart sensors need to be implemented. For instance, face recognition sensors can capture and process raw data within the camera, making them intelligent as they are not dependent on any external hardware for encoding and decoding.
“If we put AI on the edge and then perform bi-directional communication (wired or wireless), then the sensor can also benefit from it, making it a smart sensor,” says Dharmendra Kumar, IoT head, Arihant Electricals.
When vehicle systems relay sensor-generated data, drivers can receive alerts about road conditions and driving hazards, such as congested roads and highways, well before encountering these problems. When vehicle systems are connected to the roadway infrastructure, sensor-generated data can supply accurate, real-time traffic data, allowing mapping programs to plot the most efficient route, so that drivers save time and greenhouse gas emissions are minimised.
One of the biggest challenges is on the mechanical side. For vibration resistance, especially in the wheels, shock-proof sensors need to be developed. Another challenge is the lack of ecosystems due to which car companies do most of the validation for connected cars. Although a car manufacturer can create a good car, it is difficult to go for IoT and edge computing at the same time. Therefore, IoT engineers should be invited to validate them. This is a good way to create an ecosystem.
The discussion further opens up critical thoughts on the impact of add-on sensors and actuators benefitting the verification and validation process of systems in vehicles.
Deep learning to avoid chaos
Machine learning (or deep learning) is a foolproof technique for end-to-end driving in the real world and ensuring a vehicle operates well while identifying the issues. But what if something is not present in the training data? Will this technique be able to solve abrupt real-world problems?
There are two possible standard solutions:
1. Use simulations
Accurate 3D mapping of everything is important. Testing hundreds and thousands of kilometre cycles in the physical world takes over a year. However, this can be easily achieved with a simulation program overnight. But then simulators assume people drive in a civilised way, which is a bad assumption. What do you do in an unexpected situation? “Simulators like Carla from Intel may not cover all possible scenarios in real world and only give a jump start for analysing certain scenarios from real world. There is a larger cosmos outside simulations which needs to be taken care of, often called one-of or edge cases due to non-standard driving, system failure or other environmental factors,” informs Naresh Neelakantan.
2. Reinforcement learning
After loading a 3D map of the city in a simulator, the next step is to assign certain vehicles with poor driving skills. But there are perhaps more ways to badly drive a vehicle than favourably. It is unknown to what extent a bad driver can be simulated. Therefore, it is required to simulate bad driving in the real world and collect live data for better analysis. And with this, deep learning methods can pretty much pick up the complexities of a real-world traffic scenario and other factors such as light intensity, weather, stray animals, road conditions, and other vehicles present on Indian roadways. Once the risks and accidents are modelled, the traffic can be stabilised to a large degree.
Regarding deep learning applications, more datasets result in better system accuracy. Some of the famous datasets include:
Astyx HiRes
It is a combination of LiDAR, radar, and 3D object detection.
UC Berkeley DeepDrive
It has 100,000 video sequences with diverse annotations for image-level tagging, object bounding boxes, and full-frame segmentations. Google Landscape. Wide dataset of different landscapes; ideal for training a driverless car in different landscapes with varying weathers.
“This will reach a tipping point towards six or nine sigma kinds of accuracy when more Turing Machine-like AI methods coupled with quantum computing could provide deeper insights from now on,” says Naresh Neelakantan.
Where is intelligence heading?
As pointed out by Dr Rahul Kala, autonomous technology has a role in providing a safe transportation experience. For instance, a lack of awareness among truck drivers on the limit to which a person can continuously drive a vehicle causes fatigue, resulting in accidents. This is where technology comes in to prevent fatal situations.
Emphasising the role of academicians, he says academic institutes are quite active in publishing data and actively discuss ideas for solving real-world problems. “Startups, academia, and government can collaborate on projects for the future of both EVs and autonomous vehicles. In India, there are hundreds of problems—let’s solve them one-by-one,” says Dr Kala.
Integration of intelligent fast tracks into autonomous driving systems allows connected AV training for safer and faster travel. Also, deployment of fast tags facilitates quicker movement near toll plazas.
Government policies
Government agencies and test labs are coming up with new strategies to test EVs. The FAME 2 policy with regards to accelerating the adoption of EVs, which was approved in 2019 with hundred billion rupees of investment, is one such example. Thanks to such an initiative, 40,000+ EVs are currently running on Indian roads and more are expected in the years to come. Besides, 5G connectivity is also expected to play a huge role. On top of that, the government of India is already pushing forward Make in India and Atmanirbhar Bharat schemes to give Indian industry a boost.
To minimise the infrastructure bottleneck of EV charging, the Indian government, as a part of the FAME 2 policy, has planned to deploy charging stations across petrol pumps nationwide so that these are readily available for use.
Regarding policies on autonomous vehicles, there seems to be a slight hesitation in implementing them as the government is pretty much concerned about increased job losses on account of vehicle autonomy. Therefore, to be on a safe side, it is more supportive for EVs (partly due to environmental issues as well). Taking into consideration several other factors—such as Indian roads, potholes, weather, etc—it is a long way to go for autonomous vehicles to enter Indian markets.
In summary, new EV standards continue to be developed, which can be leveraged for promoting wider adoption. When it comes to intelligent vehicles, a reliable structure involving edge computing, deep learning, and IoT should be present for enhanced judgement in unforeseen situations. Finally, the government has introduced many new policies that can be leveraged for wider adoption of EVs. Despite the reluctance to adopt autonomous vehicles, India would one day inevitably have a balanced ecosystem in which both humans and autonomous vehicles co-exist.
This article is based on the panel discussion ‘Challenges faced in testing cars of the future (connected, intelligent, EV, etc) and possible solutions’ during December edition of India Technology Week 2020. The panel was chaired by Naresh Neelakantan, senior architect – System Software at Sasken Technologies Autonomous car and self-driving concept