MIT and Stanford University researchers have developed a machine-learning method that learns robot control more efficiently, achieving superior performance with less data.
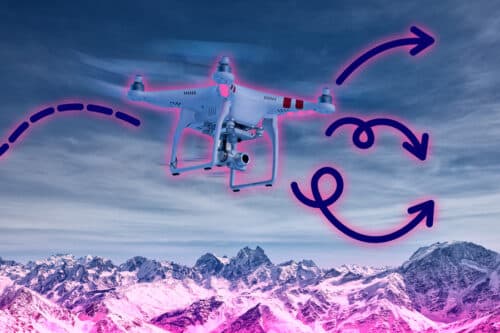
Navigating the optimal control of a robot for specific tasks remains challenging, even when researchers thoroughly understand the system’s model.
MIT and Stanford University researchers have developed a novel machine-learning method to enhance the control of robots, like drones or autonomous vehicles, in quickly changing and dynamic environments. The new technique can help autonomous vehicles handle slippery roads, assist space robots in towing objects, and enable drones to track skiers in strong winds. The method merges control theory with learning, offering hints for effective system control. This approach also requires less data, leading to quicker, improved performance in dynamic environments.
Learning a controller
A controller dictates a drone’s trajectory, adjusting rotor forces to counter winds, ensuring a stable path. Drones, evolving in position and velocity, are dynamic systems. When simple, controllers can be hand-derived, capturing a structure grounded in system physics, like the relationship among velocity, acceleration, and force. However, complexities like aerodynamic effects make manual modelling challenging. Instead, the researchers have used machine learning to analyse drone data to model these systems. But traditional methods often omit a control-based structure crucial for setting rotor speeds. After modelling, many existing techniques further require separate controller learning using data.
Identifying structure
The team have devised a technique employing machine learning to understand the dynamics model, ensuring it retains a predefined structure beneficial for system control. This structure allows direct extraction of a controller from the dynamics model, eliminating the need for a separate controller model based on additional data. When tested, the controller accurately followed desired paths, surpassing all baseline methods. Its performance was almost on par with a ground-truth controller built from the system’s precise dynamics. The team’s method efficiently used minimal data, excelling where other techniques faltered with smaller datasets. Ideal for drones or robots in fast-changing conditions, their versatile approach suits a range of dynamical systems, from robotic arms to low-gravity spacecraft.
In the future, the researchers aim to create models that offer clearer physical interpretations and can discern detailed information about a dynamical system. They believe that such advancements could result in superior controllers.