Researchers at MIT demonstrated an artificial intelligence model that can self-learn the linguistic rules and patterns of human languages.
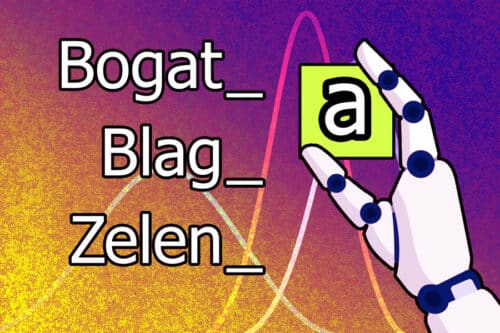
The new AI model can automatically self-learn higher-level language patterns for better performance. The researchers tested the model with problems from textbooks that featured 58 languages. The model was able to come up with answers with an accuracy rate of 60%.
The team used Bayesian Program Learning, a machine learning technique which allows the model to solve the problems by writing a computer program. They built this model using Sketch, a program synthesizer developed by Solar-Lezama, MIT. In this case, the program is the grammar as the model thinks it to be the explanation of words and meanings in a linguistic problem.
Since Sketch consumes time to process a request, the team tested one piece at a time. They gradually increased the size and the difficulty of the problems. They also ensured that the model can self-learn what “good” programs look like. The model was able to solve a complex problem in Polish after learning some general rules on simple Russian problems. This simplifies the function of the model by learning the similarities in the language and executing them.
The researchers expect their model to find unexpected solutions to the problems in their domains in the future. They could also apply the technique to more similar situations which requires higher-level knowledge across interrelated datasets. They also demonstrated that the model was able to learn a general template of phonological rules that could be applied across the problems.
“Maybe we need better methods for learning across problems. And maybe, if we can’t come up with those methods, this work can help us probe different ideas we have about what knowledge to share across problems,” says Kevin Ellis, an assistant professor of computer science at Cornell University and the lead author of the paper.