MIT researchers have developed a novel technique for analyzing unlabeled audio and visual data, enhancing machine learning models for speech recognition and object detection.
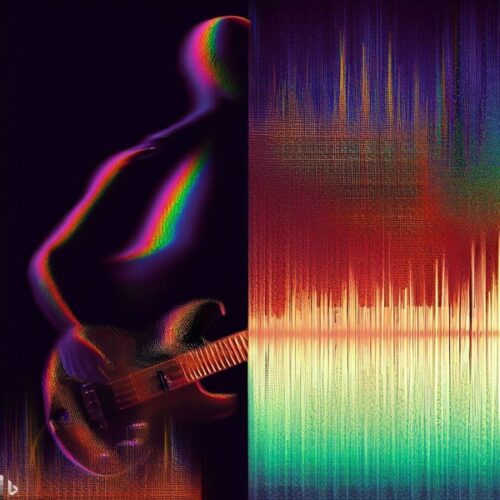
Humans often acquire knowledge through self-supervised learning due to insufficient supervision signals. Self-supervised learning is the basis for an initial model, leveraging unlabeled data. Fine-tuning can be achieved through supervised learning or reinforcement learning for specific tasks.
MIT and IBM Watson Artificial Learning (AI) Lab researchers have developed a new method to analyze unlabeled audio and visual data, improving machine learning models for speech recognition and object detection. The work merges self-supervised learning architectures, combining contrastive learning and masked data modeling. It aims to scale machine-learning tasks, such as event classification, in various data formats without annotation. This approach mimics human understanding and perception. The contrastive audio-visual masked autoencoder (CAV-MAE), technique, a neural network, learns latent representations from acoustic and visual data.
A joint and coordinated approach
CAV-MAE employs “learning by prediction” and “learning by comparison.” Masked data modeling involves masking a portion of audio-visual inputs, which are then processed by separate encoders before being reconstructed by a joint encoder/decoder. The model is trained based on the difference between the original and reconstructed data. While this approach may not fully capture video-audio associations, contrastive learning complements it by leveraging them. However, some modality-unique details, like video background, may need to be recovered.
The researchers evaluated CAV-MAE, their method without contrastive loss or a masked autoencoder, and other methods on standard datasets. The tasks included audio-visual retrieval and audio-visual event classification. Retrieval involved finding missing audio/visual components, while event classification identified actions or sounds in the data. Contrastive learning and masked data modeling complement each other. CAV-MAE outperforms previous techniques by 2% for event classification, matching models with industry-level computation. It ranks similarly to models with only contrastive loss. Incorporating multi-modal data in CAV-MAE improves single-modality representation and audio-only event classification. Multi-modal information acts as a “soft label” boost, aiding tasks like distinguishing between electric and acoustic guitars.
Bringing self-supervised audio-visual learning into our world
The researchers consider CAV-MAE a significant advancement for applications transitioning to multi-modality and audio-visual fusion. They envision its future use in action recognition for sports, education, entertainment, motor vehicles, and public safety, with potential extensions to other modalities. Although currently limited to audio-visual data, the team aims to target multimodal learning to mimic human abilities in AI development and explore other modalities.