Researchers have created a security solution for energy-intensive AI models that safeguards against two prevalent types of attacks.
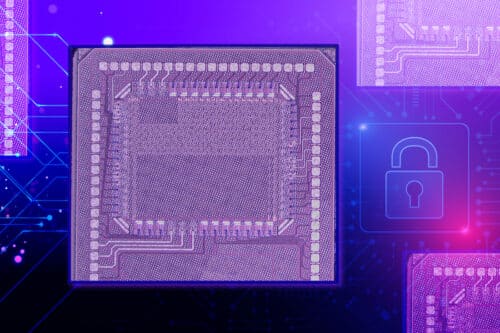
Health-monitoring apps are crucial for managing chronic diseases and achieving fitness goals. These apps utilize smartphones as the primary tool. These apps often rely on large machine-learning models that require constant data exchange between the smartphone and central servers, leading to inefficiencies and slow performance.
To address these inefficiencies, engineers employ machine-learning accelerators that reduce data transfer needs, enhancing app speed. However, these accelerators are prone to security breaches, where attackers could potentially access sensitive information like health records and financial data.
Researchers from MIT and the MIT-IBM Watson AI Lab have developed a new type of machine-learning accelerator that resists the two most prevalent attack methods, safeguarding sensitive data while maintaining efficiency. The accelerator’s design incorporates optimizations that ensure robust security with minimal impact on speed and accuracy. This technology is also applicable to AI-driven fields such as augmented reality and autonomous driving.
Trade-offs And Design Considerations
The implementation of these secure chips slightly raises the cost and reduces energy efficiency, but these are often acceptable compromises for enhanced security. The new digital in-memory compute (IMC) chip integrates security measures directly into the hardware, performing computations within the device’s memory and reducing external data transfers. This architecture not only speeds up processing but also strengthens defense against side-channel and bus-probing attacks. By encrypting data and generating unique decryption keys on the chip itself, the team has effectively blocked potential security breaches.
In testing scenarios, the researchers attempted to breach their own system using common hacking techniques but were unable to extract any sensitive information, highlighting the effectiveness of their security measures. Despite the increased energy and fabrication costs, ongoing research aims to optimize these aspects further, making the technology more feasible for widespread use.
The researchers represents a significant step forward in securing edge devices against cyber threats, crucial for the future of mobile and AI technology in various applications. The balance of security, cost, and performance will continue to be a pivotal area of focus in the development of next-generation machine-learning accelerators.