This method enables precise control of humanoid robots for a myriad of tasks, promising a new era of human-robot collaboration.
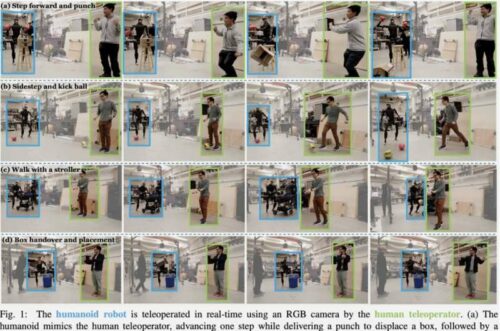
Teleoperation, the remote control of robots, is set to revolutionize various industries by enabling humans to perform a wide range of manual tasks from a distance, including those that are hazardous or intricate. Researchers at Carnegie Mellon University have recently developed a breakthrough method called Human2HumanOid (H2O), which significantly advances the teleoperation of human-sized humanoid robots. This approach, detailed in a paper on the arXiv preprint server, could pave the way for training humanoid robots in manual tasks requiring precise movements, such as playing sports, pushing trolleys, or moving boxes.
The researchers expressed optimism about the potential of humanoid robots in 2024, emphasizing the seamless integration of human cognitive skills with the versatile capabilities of humanoids. H2O marks an important step towards this integration by providing a real-time, whole-body teleoperation system using just an RGB camera. This system allows a human operator to precisely control a humanoid robot in various real-world tasks. H2O stands out from previous methods as it relies solely on an RGB camera, making it more scalable and accessible for widespread use. They highlighted the importance of human teleoperation in scaling up data collection for humanoid robots and making teleoperation more user-friendly. The H2O framework combines elements from previous studies, such as physics-based animation, motion transfer to humanoids, and teleoperation, into a cohesive system.
The framework employs reinforcement learning (RL) to enable real-time, whole-body teleoperation of humanoid robots. It starts with retargeting human motions to fit the humanoid’s physical constraints, and then uses these motions to train an RL-based motion imitator in simulation. The trained model is then transferred to the real robot without further adjustments. Despite minimal hardware requirements, H2O allows robots to perform a wide array of dynamic motions in real-time. The system’s components include a retargeting algorithm, a method for cleaning human motion data in simulations, and an RL-based model for learning new teleoperation policies. The researchers demonstrated the effectiveness of their approach in real-world tests, where a humanoid robot successfully replicated various motions, such as moving a box, kicking a ball, and pushing a stroller.
The H2O framework holds promise for training robots in a multitude of real-world tasks, from household chores to medical assistance and rescue operations. Its reliance on a standard RGB camera makes it feasible for implementation in diverse settings. The researchers plan to further improve and expand the capabilities of humanoid teleoperation, focusing on enhancing motion fidelity, addressing the sim-to-real gap, and incorporating feedback from the robot to the operator for a more immersive experience. Future developments may also include manipulation with dexterous hands and increasing the robot’s autonomy for efficient, safe, and dexterous human-robot collaboration.