New research shows that integrating real-world driving data into battery management can improve battery longevity and reliability.
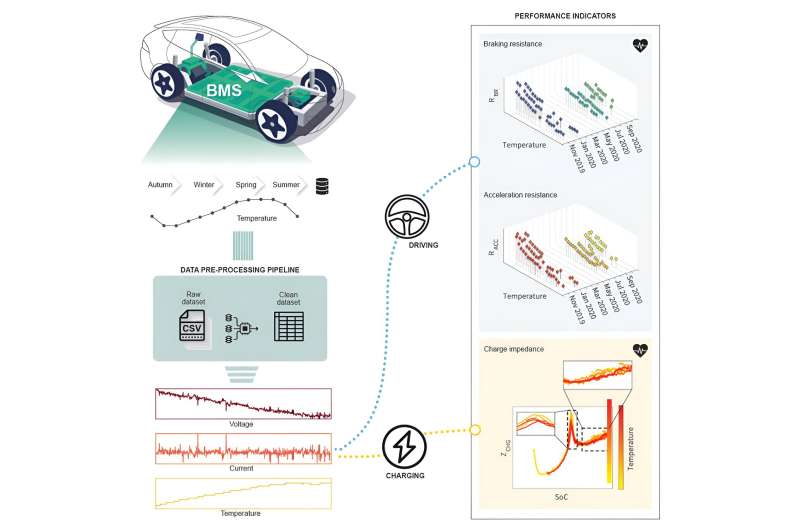
Most electric vehicles have an integrated electronic system for daily battery operations and safety. This system, known as the Battery Management System (BMS), incorporates software with algorithms that track the health of the robust lithium-ion battery pack.
Researchers at Stanford University have developed an algorithm to demonstrate the gap between controlled laboratory testing and actual road experience. Algorithms developed using unrealistic driving data will likely need to be more accurate in the field. The team aims to enhance battery pack longevity by crafting algorithms informed by genuine real-world data.
Driving styles
Battery management systems in electric cars on the road consistently collect data during braking, acceleration, deceleration, and charging. Whether driving is aggressive or partially charged in their vehicle, varied driving and charging habits lead to distinct battery degradation trajectories. Yet, this field data type needs to be incorporated into traditional battery algorithms. For research, the team was granted approximately 3,750 hours of BMS driving data by Volkswagen, which was gathered from an all-electric Audi e-tron SUV. The vehicle was driven in the San Francisco Bay Area over a year, from November 2019 to October 2020.
Energy and Power
Utilizing the field data from Audi, the researchers have determined the electrical resistance within the battery pack over a year, which allowed them to evaluate two primary battery metrics: energy and power. The team derived resistance values by observing sudden shifts in the battery’s current and voltage using data from 529 acceleration instances and 392 braking occurrences throughout the year. They also deduced impedance, a resistance metric during charging, by examining 53 charging events. The researchers found that electrical resistance diminished in colder periods but ascended during spring and summer, indicating enhanced battery health with rising temperatures.
The lab vs. the road
Automakers typically depend on traditional BMS algorithms conceived under controlled lab settings, wherein these algorithms, often developed using machine learning (ML), track performance metrics from a singular 4-volt battery cell that consistently charges and discharges at a fixed temperature until depletion. In contrast, the field data from Audi was derived from a 396-volt battery setup of 384 cells.
The researchers aim to forge algorithms that guide drivers in prolonging the lifespan of the battery pack, the car’s priciest component. This could involve sending alerts to drivers regarding excessive fast charging or overly aggressive acceleration. Field data offers invaluable insights to enhance the resilience and efficacy of BMS algorithms.
Reference: Gabriele Pozzato et al, Analysis and key findings from real-world electric vehicle field data, Joule (2023). DOI: 10.1016/j.joule.2023.07.018