Researchers from Cambridge University have developed a machine learning algorithm that could help prolonging the battery life in e-vehicles through driving patterns.
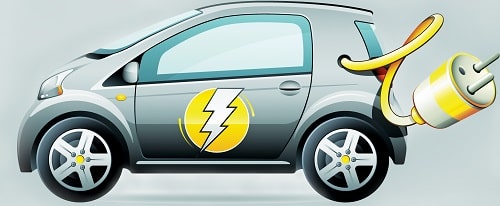
The team has developed a non-invasive probe that sends high-dimensional electrical pulses into a battery. It then measures the response and provides a series of ‘biomarkers’ of battery health. This method on the battery doesn’t cause it to degrade any further.
The electrical signals from the battery are then converted into a description of the state of the battery. The extracted data is then fed into a machine learning algorithm. This algorithm was capable of predicting the patterns on how the battery would respond in the next charge-discharge cycle. The team tested the same with 88 commercial batteries and the algorithm required only the data from the previous usage of the battery. This experiment was mainly focused on Lithium Cobalt Oxide (LCO) cells. However, the same method is applicable for different types of rechargeable batteries used in electric vehicles.
The team is currently working with the manufacturers to take this to the next level. The primary aim is to accelerate the development of safer and long lasting rechargeable batteries. They are also working on improvising the algorithm to develop optimal fast charging protocol. This protocol aims at reducing the charging times taken by the electric vehicles without causing further degradation. They also say that their method will also benefit large logistics businesses that engage large fleets of electric vehicles.
“Most of us will replace our phones well before the battery degrades to the point that it’s unusable, but for cars, the batteries need to last for five, ten years or more,” said Dr. Alpha Lee, who led the research. “Battery capacity can change drastically over that time, so we wanted to come up with a better way of checking battery health.”