Stanford engineers created a more efficient AI chip that could bring the power of AI into tiny edge devices.
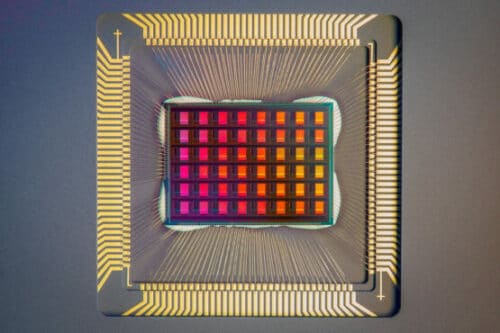
Stanford University engineers have created a chip that does AI processing with in-built memory, eliminating the compute and memory units. This “compute-in-memory” (CIM) chip is called NeuRRAM and works with a limited battery power.
The NeuRRAm uses the resistive random-access memory (RRAM) technology. This memory tech enables the chip to retain data even when turned off. Also, the RRAM can store large AI models in a small area footprint at minimal power consumption, making these the perfect low-power edge devices.
“This is one of the first instances to integrate a lot of memory right onto the neural network chip and present all benchmark results through hardware measurements,” said Wong, co-senior author of the Nature paper.
The designed architecture of the NeuRRAM enables the chip to perform analog in-memory computation at lower power consumption and in a compact area footprint. It was designed at the University of California, San Diego, in collaboration with Gert Cauwenberghs lab, who pioneered low-power neuromorphic hardware design. This architecture also allows the user to reconfigure in dataflow directions. It also supports various AI workload mapping strategies and is capable of working with various AI algorithms.
The team tested the NeuRRAM with different tasks to know its ability and accuracy. The result showed 99% accuracy in letter recognition from MNIST dataset, 84.7% accurate on Google speech command recognition, 70% reduction in image reconstruction error on Bayesian image recovery task and 85.7% accuracy on image classification from the CIFAR-10 dataset. This chip is expected to fulfill most of the AI needs in almost every sector.
“By having these kinds of smart electronics that can be placed almost anywhere, you can monitor the changing world and be part of the solution,” Wong said. “These chips could be used to solve all kinds of problems from climate change to food security.”