As the push for more advanced AI continues, Lincoln Laboratory is discovering methods to decrease energy consumption, enhance training efficiency, and clarify energy usage.
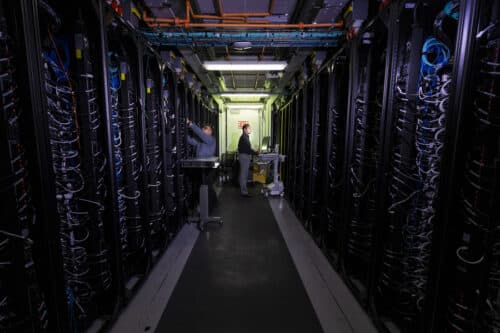
Google’s recent move to display carbon emissions alongside flight prices is an initiative to promote sustainable choices among travellers. However, the tech industry, especially in artificial intelligence (AI), hasn’t shown similar transparency, even though its carbon footprint surpasses that of the entire aviation sector. The rise of massive AI models like ChatGPT signifies a trend towards large-scale artificial intelligence, raising concerns about future energy consumption. Predictions suggest that by 2030, data centres could account for 21% of global electricity use.
Enter the MIT Lincoln Laboratory Supercomputing Center (LLSC). They’re devising energy-saving strategies for data centres, from simple interventions like hardware power-capping to sophisticated tools for halting inefficient AI training. Notably, these methods barely affect model outcomes. “The secrecy surrounding energy usage in AI research needs to end. We’re initiating this change and hope others follow,” comments Vijay Gadepally, a senior LLSC staff.
Innovations to Reduce GPU Energy Use
Recognising the increasing energy demands of AI operations, the researchers have actively championed green computing. For instance, training AI models relies heavily on graphics processing units (GPUs), which are notably energy-intensive. GPT-3’s training, for example, consumed energy equivalent to the monthly consumption of 1,450 average US households. By utilising built-in power restrictions for GPUs, the LLSC team managed to cut energy consumption by around 12-15%. The slight drawback is a minimal increase in task completion time, which is negligible in the grand scheme of things.
Implementing these power limits has the added benefit of reducing GPU temperature by around 30°F, easing the cooling system’s load and potentially extending hardware longevity. The LLSC collaborated with Northeastern University experts to devise a comprehensive framework for assessing the carbon footprint of high-end computing systems. This tool empowers industry players to gauge their sustainability levels and inform future decisions. They also address the energy drain from the model-training process. They’ve crafted a predictive tool that efficiently stops underperforming AI models early in their training, achieving an impressive 80% energy saving. The team has developed an optimiser that matches AI models with the most energy-efficient hardware, reducing energy use by 10-20% without compromising performance.
AI conferences are slowly highlighting ethical concerns around climate impact. Recognising this gap, the team aims to equip AI developers with tools for informed energy choices, starting with a partnership with the US Air Force to optimise their vast network of data centres. “We’re empowering AI developers to make greener choices,” asserts Gadepally. “We hope to set a benchmark for sustainable AI development.”