This technique allows for more compact designs, reduced manufacturing costs, and enhanced computing speeds.
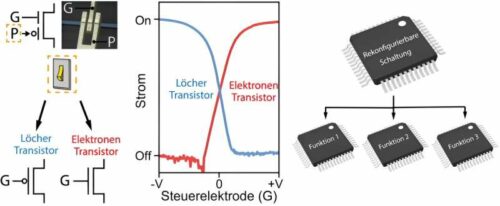
In a development at TU Wien, researchers have revolutionized the design of electronic circuits by creating intelligent, controllable transistors that can be flexibly switched between different tasks. This innovation allows for the same functionality to be accommodated on less chip space, resulting in lower manufacturing costs, reduced energy consumption, and faster computing speeds. Traditionally, microelectronics has relied on doped semiconductors, where the function of transistors is fixed during manufacture. However, the TU Wien team’s new transistor concept, known as “electrostatic doping,” eliminates the need for doped material. Instead, the behavior of charge carriers is controlled by electric fields, with electric charge introduced into the transistor via an additional electrode.
This approach enables the reconfiguration of a circuit’s function according to specific requirements, allowing for more compact and versatile designs. For example, using this technology, an addition circuit can be created from two compact XOR links, a feat that would require two separate circuits with conventional technology. The research team overcame the major challenge of operating components either by transporting electrons or holes with the same switching properties. This innovation holds great promise for the chip industry, as it allows for increased functionality on the same surface area, reducing the need for transferring information between different blocks within a chip, which is both time-consuming and energy-intensive.
The research group is already collaborating with companies in the chip industry, and while the transition to this new approach is significant, it does not require new materials or processes, utilizing familiar materials like silicon and germanium. As miniaturization in microelectronics approaches its natural limits, intelligent, reconfigurable components offer an exciting option for the future of computing. They could pave the way for intelligent, self-learning, or even neural computer systems that can adapt their function to the requirement profile, enabling calculations to be performed as quickly and energy-efficiently as possible.