The neuromorphic biosensors, equipped to self-train and adapt for multiple uses, are poised to transform healthcare technology through fast and energy-saving on-chip learning processes.
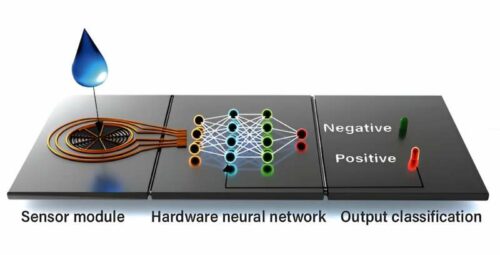
Computers modelled after the human brain structure, known as neuromorphic computers, have the potential to transform the healthcare devices of the future. However, their adoption needs to be improved due to the requirement of external training software, which can be both energy-draining and time-consuming.
Researchers from Eindhoven University of Technology and Northwestern University in the U.S. have developed a new neuromorphic biosensor with integrated learning capabilities, eliminating external training. Demonstrating its efficacy, the team utilised this biosensor to identify cystic fibrosis by analysing sweat samples in a proof-of-concept experiment.
Goodbye to external software
The obstacle that the team have overcome pertains to the practical deployment of neuromorphic technologies in healthcare devices. These technologies need to have minimal power consumption, be able to interface with a sensor, and be easily trainable for utilisation. The first two requirements can be addressed using organic-based electronics. However, the central challenge lies in the training aspect. Traditionally, the neural network of a neuromorphic chip would be trained through external software, a procedure that tends to be both time-consuming and not energy-efficient.
Searching for chloride anions
The researchers conducted cystic fibrosis tests to evaluate the innovative chip, a genetic disorder affecting vital organs. Utilising the established sweat test method, where high chloride anion levels indicate the disease, the team found a practical case study to verify their on-chip learning sensor. The neuromorphic biosensor, comprising a sensor module, neural network hardware, and an output classification segment, identified ion concentrations in sweat samples through ion-selective electrodes. The chip then processed this data, displaying the results as a green or red light, representing negative or positive results, respectively.
Training at the ‘data gym’
Before using the chip to analyse primary sweat samples, the neural network underwent supervised training or a stint in the data gym. This constitutes the research’s significant breakthrough, the chip’s ability to self-train for particular tasks, such as identifying cystic fibrosis from sweat samples, without needing external software or further interventions.
The ease of retraining
Furthermore, the versatility of the newly developed chip allows it to be repurposed for different applications, even post-training. This innovative on-chip learning strategy paves the way for creating personalised implantable neural networks that are user-trained, leveraging data directly sourced from the individual.Â
Reference: E. R. W. van Doremaele et al, A retrainable neuromorphic biosensor for on-chip learning and classification, Nature Electronics (2023). DOI: 10.1038/s41928-023-01020-z