Researchers at Stanford University have developed Sonicverse, an artificial environment designed to train AI agents or robots using both visual and auditory elements.
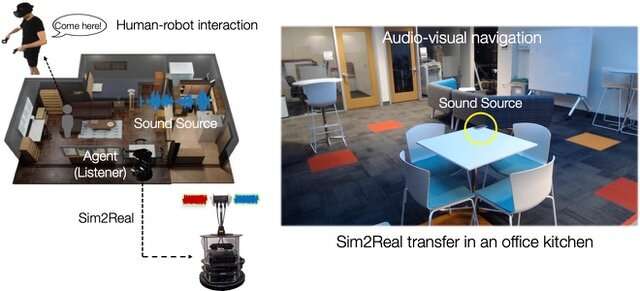
Artificial Intelligence (AI) robots are becoming more advanced and are being used in various real-world settings such as malls, airports, and hospitals. They may also help with household chores and office tasks. However, before deploying robots in real environments, their AI algorithms must be trained and tested in simulated settings. Currently, few platforms consider the sounds robots encounter during tasks.
A team of researchers at Stanford University have created Sonicverse, a simulated environment for training AI agents or robots with visual and auditory components. The team aimed to create a multisensory simulation platform for training household agents capable of seeing and hearing, with realistic audio-visual integration.
The team have developed Sonicverse, an advanced simulation platform that encompasses visual and auditory aspects of various environments. By accurately modelling the visual elements and corresponding sounds that an agent would encounter during exploration, the researchers aimed to enhance robot training in virtual spaces that closely resemble the real world. This approach aimed to improve the subsequent performance of robots when deployed in real-life scenarios.
The researchers employed Sonicverse to train a simulated TurtleBot in navigating through an indoor space and reaching a designated location without encountering obstacles. Subsequently, they transferred the AI trained in the simulations to a physical TurtleBot, assessing its audio-visual navigation skills within an office environment. Sonicverse’s realism was successfully demonstrated through sim-to-real transfer, a feat unmatched by other audio-visual simulators. In other words, the researchers showcased that an agent trained with Sonicverse effectively carries out audio-visual navigation in real-world settings, including office kitchens. The researchers’ test results exhibit great promise, indicating that their simulation platform has the potential to enhance the effectiveness of training robots for real-world tasks by incorporating visual and auditory stimuli.
Embodied learning utilising multiple modalities holds tremendous potential for unlocking a wide range of applications in future household robots. The team aimed to integrate multisensory object assets into the simulator. The researchers believe that this integration will enable modelling multisensory signals at both the spatial and object levels, while also incorporating additional sensory modalities such as tactile sensing.
Reference: Ruohan Gao et al, Sonicverse: A Multisensory Simulation Platform for Embodied Household Agents that See and Hear, arXiv (2023). DOI: 10.48550/arxiv.2306.00923