Discover how this technology is breaking barriers to equip drones, rovers, and robots with the ability to learn from their environments continuously, ensuring they adapt in real time to unforeseen challenges.
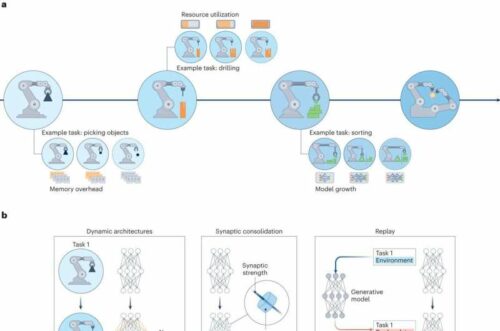
In the rapidly evolving domain of artificial intelligence (AI) and autonomous devices, the concept of “lifelong learning” transcends conventional learning apps, embracing a more intricate meaning. It signifies a device’s capacity to incessantly learn from and adapt to its environment autonomously and in real-time. This capability is pivotal for the advancement of cutting-edge technologies like automated delivery drones, self-driving cars, extraplanetary rovers, and robots tasked with hazardous jobs.
At the heart of these innovations are scientists working tirelessly to develop algorithms that enable such autonomous learning. However, a significant hurdle lies in ensuring the specialized AI accelerators—chips necessary for running these advanced algorithms—can keep pace. Angel Yanguas-Gil, a researcher at the U.S. Department of Energy’s Argonne National Laboratory, is at the forefront of tackling these challenges. His work, integral to Argonne’s Microelectronics Initiative, was recently featured in a Nature Electronics paper that delves into the programming and hardware obstacles AI-driven devices encounter and potential strategies for overcoming them. Traditional AI operates on a training and inference model, where AI systems are pre-trained offline and then deployed, unable to learn from new data or experiences.
This model falls short in dynamic environments where devices may encounter unforeseen challenges. Yanguas-Gil emphasizes the necessity for devices like planetary rovers to adapt in real-time to new data without the need for retraining from scratch—a process impractical due to time constraints and the devices’ operational contexts. The pursuit of real-time learning capabilities introduces the need for more sophisticated algorithms, which, in turn, demand more from the hardware accelerators in terms of energy, memory, and flexibility. These accelerators must be compact, energy-efficient, and capable of evolving resource utilization over time to accommodate new learning without forgetting previous knowledge—a phenomenon known as “catastrophic forgetting.”
The team identifies several key features required for AI accelerators to support lifelong learning: on-device learning capability to eliminate reliance on external data sources, adaptability in resource allocation, model recoverability to maintain performance despite ongoing learning, and the ability to integrate new knowledge with existing data effectively. Addressing these requirements poses a formidable challenge, one that researchers are only beginning to tackle, paving the way for the next generation of autonomous devices capable of continuous, real-time learning.