Researchers have developed a new neuro-vector-symbolic architecture that solves abstract reasoning and analogy-related problems.
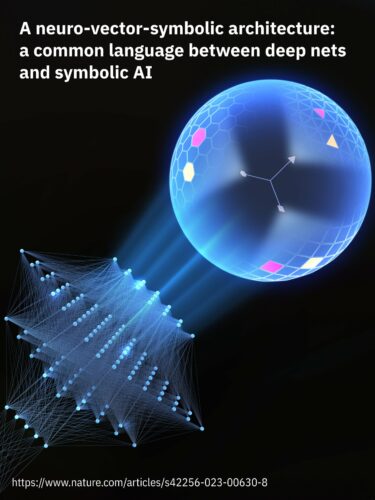
System architecture refers to the way individual components of a particular system are organized and connected to one another in order to function together as a cohesive unit. With these booming advancements in the field of artificial intelligence and machine learning, data and information has become more demanding than ever before. The quest to make AI more reasonable can be dealt with by training these architectures.
Researchers at IBM Research Zürich and ETH Zürich have recently created a new architecture that combines two of the most renowned artificial intelligence approaches, namely deep neural networks and vector-symbolic models. This combination was previously applied to few-shot learning as well as few-shot continual learning tasks, achieving state-of-the-art accuracy with lower computational complexity.
In their work, researchers focused on solving visual abstract reasoning tasks, specifically, the widely used IQ tests known as Raven’s progressive matrices. To solve Raven’s progressive matrices, respondents need to correctly identify the missing items in given sets among a few possible choices. This requires advanced reasoning capabilities, such as being able to detect abstract relationships between objects, which could be related to their shape, size, color, or other features.
Researchers developed two key enablers of our architecture. The first is the use of a novel neural network training method as a flexible means of representation learning over VSA. The second is a method to attain proper VSA transformations such that exhaustive probability computations and searches can be substituted by simpler algebraic operations in the VSA vector space.
In initial evaluations, the architecture attained very promising results, solving Raven’s progressive matrices faster and more efficiently than other architectures developed in the past. Specifically, it performed better than both state-of-the-art deep neural networks and neuro-symbolic AI approaches, achieving new record accuracies of 87.7% on the RAVEN dataset and 88.1% on the I-RAVEN dataset.
In contrast with existing architectures, NVSA can perform extensive probabilistic calculations in a single vector operation. This in turn allows it to solve abstract reasoning and analogy-related problems, such as Raven’s progressive matrices, faster and more accurately than other AI approaches based on deep neural networks or VSAs alone. The new architecture created by this team has so far proved to be highly promising for efficiently and rapidly solving complex reasoning tasks.
Reference : Michael Hersche et al, A neuro-vector-symbolic architecture for solving Raven’s progressive matrices, Nature Machine Intelligence (2023). DOI: 10.1038/s42256-023-00630-8