With the help of judgment by AI and its suggestions, an undesirable result can be transformed into a more desirable one
While AI technologies can automatically make decisions from a given data, it often reaches conclusions through unclear and potentially problematic means (known as the “black box” phenomenon).
Now, Fujitsu Laboratories and Hokkaido University have developed a new technology based on the principle of “explainable AI” that automatically presents users with steps needed to achieve the desired outcome based on AI results about data. “Explainable AI” represents an area of increasing interest in the field of artificial intelligence and machine learning.
Although certain techniques can also provide hypothetical improvements when an undesirable outcome occurs for individual items, these do not provide any concrete steps to improve. Explainable AI provides individual reasons for these decisions.
For instance, if an AI that makes judgments about the subject’s health status and determines that a person is unhealthy, the new technology can be applied to first explain the reason for the outcome from health examination data like height, weight and blood pressure. Then, the new technology can additionally offer the user targeted suggestions about the best way to become healthy, identifying the interaction among a large number of complicated medical checkups from past data and showing specific improvement steps that take into account the feasibility and difficulty of implementation.
Developmental Background
Deep learning technologies widely used in AI systems utilise face recognition and automatic driving to make various decisions based on a large amount of data using a kind of black box predictive model. In the future, ensuring the transparency and reliability of AI systems will become an important issue for AI to make important decisions and proposals for society. This need has led to increased interest and research into “explainable AI” technologies.
In the case of medical checkups, AI can successfully determine the level of risk of illness based on data like weight and muscle mass. Also, explainable AI presents the attributes that serve as a basis for judgment.
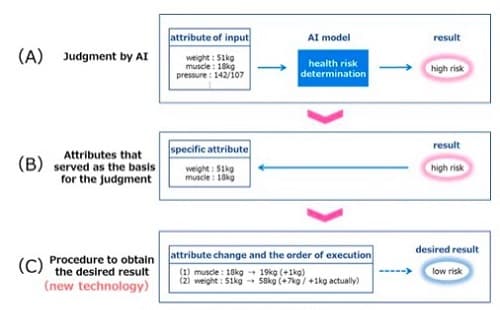
Because AI determines that high health risks are based on the attributes of the input data, it’s possible to change the attribute values to get the desired results of low health risks.
Issues
In order to achieve the desired results in AI automated decisions, it is necessary not only to present the attributes that need to be changed, but also to present the attributes that can be changed with as little effort as is practical.
Once again taking the case of medical checkups, if one wants to change the outcome of the AI’s decision from a high-risk status to low risk status, achieving it with less effort may seem to increase muscle mass. But it is unrealistic to increase one’s muscle mass alone without changing one’s weight. So increasing weight and muscle mass simultaneously is a more realistic solution.
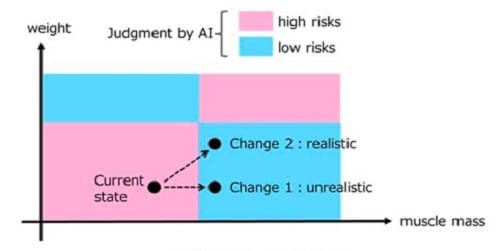
The newly developed technology is based on the concept of counterfactual explanation and presents the action in attribute change and the order of execution as a procedure. While avoiding unrealistic changes through the analysis of past cases, the AI estimates the effects of attribute value changes on other attribute values, such as causality, and calculates the amount that the user has to change based on this, enabling the presentation of actions that will achieve optimal results in the proper order and with the least effort.
So, if one has to add 1 kg of muscle mass and 7 kg to their body weight to reduce the risk in the input attribute to obtain the desired result, then it’s possible to estimate the relationship by analysing the interaction between the muscle mass and the bodyweight in advance. That means that if one adds 1 kg of muscle mass, the bodyweight will increase by 6 kg. In this case, out of the additional 7 kg required for weight change, the amount of change required after the muscle mass change is just 1 kg. In other words, the amount of change one has to make is to add 1 kg of muscle mass and 1 kg of weight, so one can get the desired result with less effort than the order changing their weight first.
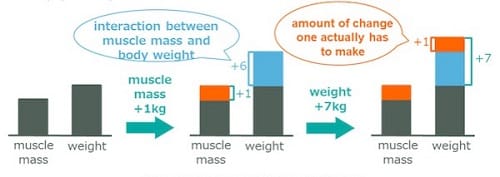
Effects
Using the counterfactual explanation AI technology, three types of data sets have been verified that are used in the following use cases: diabetes, loan credit screening and wine evaluation. By combining three key algorithms for machine learning – Logistic Regression, Random Forest and Multi-Layer Perceptron with the newly developed techniques, it is possible to identify the appropriate actions and sequence to change the prediction to the desired result with less effort.
Using this technology, when an undesirable result is expected in the automatic judgment by AI, the actions required to change the result to a more desirable one can be presented. This will allow for the application of AI to be expanded not only to judgment but also to support improvements in human behaviour.
This new technology offers the potential to improve the transparency and reliability of decisions made by AI, allowing more people in the future to interact with technologies that utilise AI with a sense of trust and peace of mind.