ACCEL combines optical with electronic computation with scalability, nonlinearity and flexibility in one chip.
Scientists at Tsinghua University in China have created an entirely analog photoelectronic chip that fuses diffractive optical analog computing (OAC) and electronic analog computing (EAC) , delivering ultrafast and highly energy efficient computer vision processing, outperforming traditional digital processors.
Photonic computing offers a path to more rapid and power-efficient processing for vision data. But its practical deployment has been hindered by complex optical nonlinearities, significant energy demands of analog-to-digital converters (ADCs), and sensitivity to noise and errors.
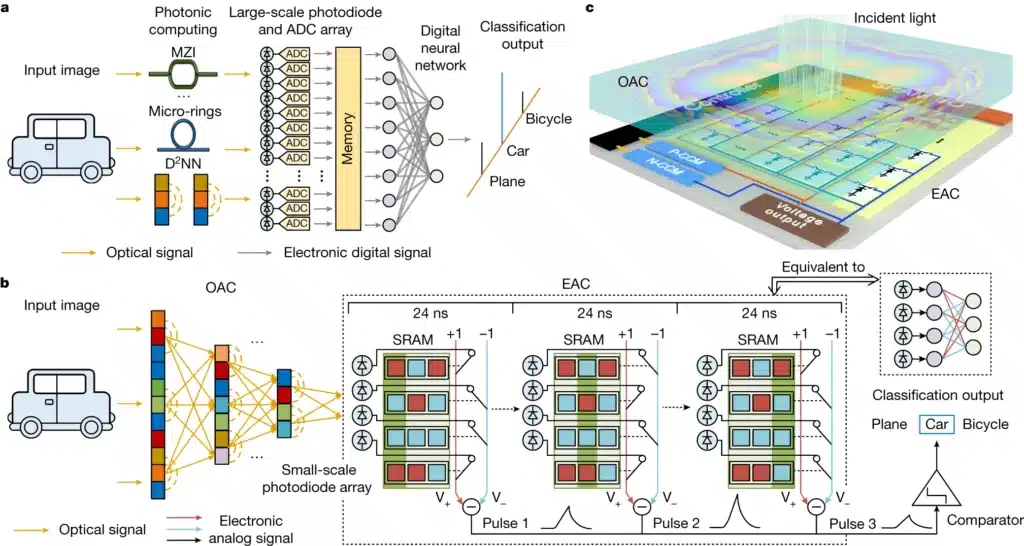
The proposed all-analog chip combining electronic and light computing (ACCEL) chip merges electronic and photonic computing, achieving energy efficiency of 74.8 peta-operations per second per watt and a processing speed of 4.6 peta-operations per second.
These metrics are orders of magnitude above the capabilities of current top-tier computing processors. Using diffractive optical computing for initial feature extraction, ACCEL leverages the ensuing photocurrents for additional processing within the same analog chip, circumventing the need for ADCs and thus reducing latency to just 72 ns per frame.
Optimisations in optoelectronic computing alongside adaptive training allow ACCEL to reach classification accuracy of 85.5% for Fashion-MNIST, a dataset of fashion articles images for benchmarking machine learning algorithms.
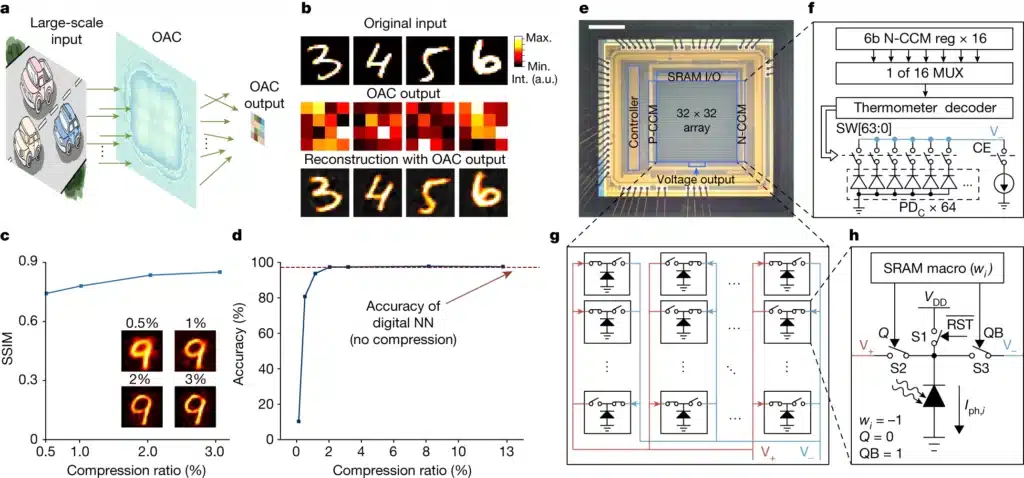
It achieves 82.0% accuracy in the 3-class ImageNet challenge. ImageNet is a large visual database used for visual object recognition software research. It also achieves 92.6% accuracy for time-lapse video recognition tasks, even under low illumination (0.14 fJ μm−2 per frame).Â
The chip is suitable for a wide array of uses, including wearable technology, autonomous vehicles, and industrial quality checks. The team aims to investigate more advanced photoelectronic computing architectures to tackle broader computer vision applications and to adapt this technology to novel AI algorithms, including large language models (LLM).