An electronic tattoo that can be comfortably worn on the wrist for hours and provides continuous blood pressure measures with an accuracy level that surpasses practically all solutions on the market today has been developed by researchers at Texas A&M University and The University of Texas at Austin.
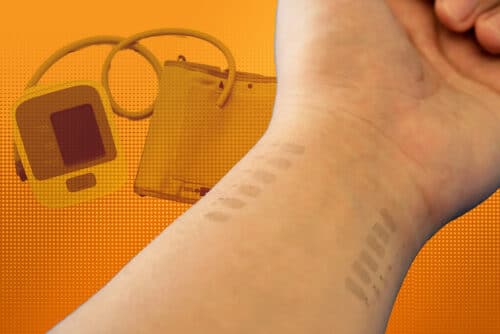
“Blood pressure is the most important vital sign you can measure, but the methods to do it outside of the clinic passively, without a cuff, are very limited,” said Deji Akinwande, a professor in the Department of Electrical and Computer Engineering at UT Austin and one of the co-leaders of the project, which is documented in a new paper published today in Nature Nanotechnology.
This electronic tattoo contains graphene, one of the toughest and thinnest materials ever created. Although the atoms are neatly organised into thin layers, it is comparable to the graphite found in pencils. As a means of mobile blood pressure monitoring, e-tattoos make sense since the sensors are enclosed in a sticky, elastic material that is both secure and comfortable to wear for extended periods of time.
“The sensor for the tattoo is weightless and unobtrusive. You place it there. You don’t even see it, and it doesn’t move,” said Roozbeh Jafari, a professor of biomedical engineering, computer science and electrical engineering at Texas A&M and the other co-leader of the project. “You need the sensor to stay in the same place because if you happen to move it around, the measurements are going to be different.”
Also, blood pressure readings are made possible by the e-continuous tattoo’s monitoring system in a variety of circumstances, including times of high stress, when sleeping, working out, etc. More measures can be delivered by this gadget than any other up to this point.
The device measures the bioimpedance of the body by applying an electrical current to the skin and observing the body’s response. Bioimpedance alterations and variations in blood pressure related to variations in blood volume are correlated. To obtain correct blood pressure measurements, the team had to develop a machine learning algorithm to examine the correlation because it is not particularly clear.
“All this data can help create a digital twin to model the human body, to predict and show how it might react and respond to treatments over time,” Akinwande said.