Scientists at Forschungszentrum Jülich published a guide for memristor hardware design to understand the various physical and chemical effects in memristors and project the influence of these effects on the switching properties of memristive cells and their reliability
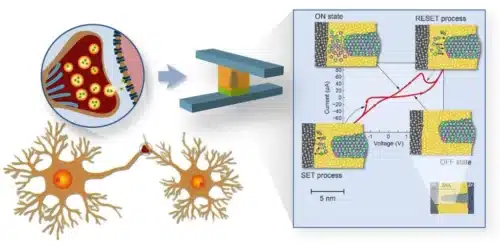
The working of memristor cells is very unique, the varying electrical resistance and can be set and reset again by applying an external voltage. The resistance value varies by the movement of oxygen ions and if these ions move out of the metal oxide layer then electrical resistance drops drastically making the material more conductive. Hence this variation in resistance value can be used for data storage. Scientists have been working for more than 15 years to develop a special data storage device that has properties similar to synapses in the human brain.
The processes that occur in cells are very difficult to analyze and vary depending on the material system. Hence, the three researchers from the Jülich Peter Grünberg Institute Prof. Regina Dittmann, Dr. Stephan Menzel, and Prof. Rainer Waser, have therefore compiled their research results in a detailed review article, “Nanoionic memristive phenomena in metal oxides: the valence change mechanism.”
“If you look at current research activities in the field of neuromorphic memristor circuits, they are often based on empirical approaches to material optimization,” said Rainer Waser, director at the Peter Grünberg Institute. “Our goal with our review article is to give researchers something in order to work with to enable insight-driven material optimization.” The team of authors worked on the approximately 200-page article for ten years and naturally had to keep incorporating advances in knowledge.
The “Roadmap of Neuromorphic Computing and Engineering,” which was published in May 2022, reveals how utilizing neuromorphic computing can reduce the huge amount of energy consumption in the IT industry. The involvement of neuromorphic circuits in the field of artificial intelligence, such as pattern recognition or speech recognition can be useful in a special way. Memristors are capable of processing massive amounts of data without transporting them between processor and memory. This could save energy efficiency of the artificial neural networks. The Memristor cells can also be interconnected to permit neural networks to learn locally. Thus, without sending data via the cloud, monitoring and controlling processes can be performed.