Researchers have developed a model to mimic the brain’s ability of making and retaining more memories than the actual brain.
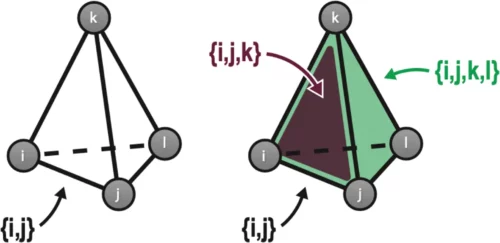
Researchers have been working on creating computer models that could mimic the working of the brain. These computer models play an important role in understanding the brain’s process of making and retaining memories and other intricate information. The intricate interplay of electrical and biochemical signals, as well as the web of connections between neurons and other cell types, creates the infrastructure for memories to be formed. Despite this, encoding the complex biology of the brain into a computer model for further study has proven to be a difficult task due to the limited understanding of the underlying biology of the brain.
Researchers at the Okinawa Institute of Science and Technology (OIST) have made improvements to a widely utilized computer model of memory, known as a Hopfield network, by incorporating insights from biology. Hopfield networks store memories as patterns of weighted connections between different neurons in the system. The network is “trained” to encode these patterns, then researchers can test its memory of them by presenting a series of blurry or incomplete patterns and seeing if the network can recognize them as one it already knows.
In classical Hopfield networks, however, neurons in the model reciprocally connect to other neurons in the network to form a series of what are called “pairwise” connections. Pairwise connections represent how two neurons connect at a synapse, a connection point between two neurons in the brain. But in reality, neurons have intricate branched structures called dendrites that provide multiple points for connection, so the brain relies on a much more complex arrangement of synapses to get its cognitive jobs done.
Researchers created a modified Hopfield network in which not just pairs of neurons but sets of three, four, or more neurons could link up too, such as might occur in the brain through astrocytes and dendritic trees. The new network allowed these so-called “set-wise” connections; overall it contained the same total number of connections as before. Researchers found that a network containing a mix of both pairwise and set-wise connections performed best and retained the highest number of memories. They estimate it works more than twice as well as a traditional Hopfield network.