With optimised techniques and machine learning, underwater robots can be created that mimic the movement and manoeuvrability of fish
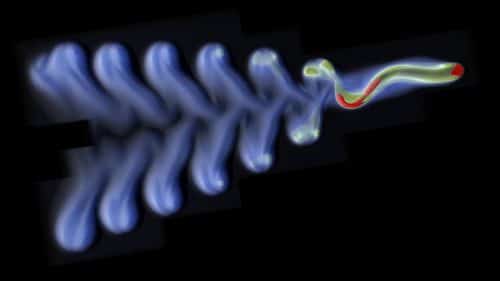
The ocean is a place whose large portions are yet to be explored. Advanced communication technology is required to tackle the unpredictable nature of oceans. With developments in additive manufacturing, optimisation techniques and machine learning, underwater robots can be created that mimic the movement and manoeuvrability of fish.
Therefore, a research team from the Massachusetts Institute of Technology (MIT) is using numerical simulation approaches to design underwater devices that have high degrees of freedom due to their fish-like, deformable fins.
To achieve their goal, the researchers are studying the simulations of a fin that will help them better understand the dynamics between the fluid and fish’s structure of soft, flexible fins as it moves through a fluid flow. As a result, they can determine how fin shape deformations can harm or improve swimming performance.
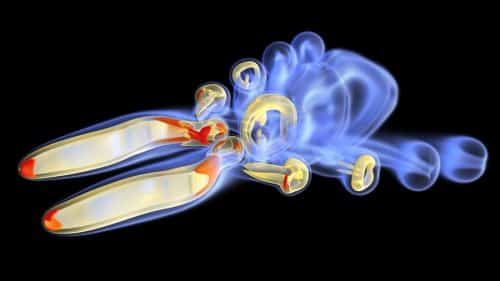
“By developing accurate numerical techniques and scalable parallel implementations, we can use supercomputers to resolve what exactly happens at the interface between the flow and the structure,” says Wim van Rees, the ABS Career Development Professor at MIT.
The tool aims to help engineers and developers to develop agile underwater robots that can navigate their paths in a better way and perform their assigned operations.
“We can use this optimisation and AI to do inverse design inside the whole parameter space and create smart, adaptable devices from scratch, or use accurate individual simulations to identify the physical principles that determine why one shape performs better than another,” explains van Rees.
Robust algorithms for numerous robotic vehicles
With a vision similar to that of Wim van Rees, Principal Research Scientist Michael Benjamin wishes to improve the manoeuvrability of underwater vehicles. He aims to optimise his 2006 open-source software project by adding obstacle-avoidance algorithms that would enable vehicles to communicate with one another.
He and his team have developed algorithms with which each vehicle can periodically communicate its location to other vehicles nearby, enabling these vehicles to disperse and scan the ocean collectively. Based on COLREGS — or “Collision Regulations”, these rules determine which vehicles have the “right of way” when crossing paths, thus avoiding any collision.
The algorithm developed by the research team ranks specific manoeuvres on a scale from zero to 100: zero for a direct collision, while 100 means complete avoidance of collision.
Braving the unpredictable environment
Uneven fluid dynamics, changing tides, weather patterns and climate change make the ocean an unpredictable environment that is different from one moment to the next. This ever-changing nature of the ocean environment can make forecasting incredibly difficult.
Although dynamical system models do help make predictions for ocean environments, they have their limitations.
“You can’t account for every molecule of water in the ocean when developing models. The resolution and accuracy of models and ocean measurements are limited. There could be a model data point every kilometre or 10 kilometres. That can have a large impact on the accuracy of your prediction,” says Pierre Lermusiaux, the Nam Pyo Suh Professor at MIT.
Assisting him is graduate student Abhinav Gupta who has helped in developing a new machine-learning framework to make up for the lack of resolution or accuracy in these models. Their algorithm introduces time delays in existing approximate models to improve predictive capabilities.
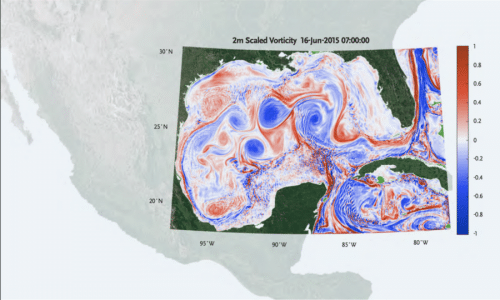
“Things in the natural world don’t happen instantaneously; however, all the prevalent models assume things are happening in real-time,” says Gupta. “To make an approximate model more accurate, the machine learning and data you are inputting into the equation need to represent the effects of past states on the future prediction.”
The team’s “neural closure model,” which accounts for these delays, could potentially lead to improved predictions for things such as a Loop Current eddy hitting an oil rig in the Gulf of Mexico, or the amount of phytoplankton in a given part of the ocean.
As computing technologies such as Gupta and Lermusiaux’s neural closure model continue to improve and advance, researchers can start unlocking more of the ocean’s mysteries and develop solutions to the many challenges our oceans face.