Researchers at the University of California, Davis College of Engineering, have used machine learning to predict solar cell behaviour and discover high-efficiency materials with fewer experiments.
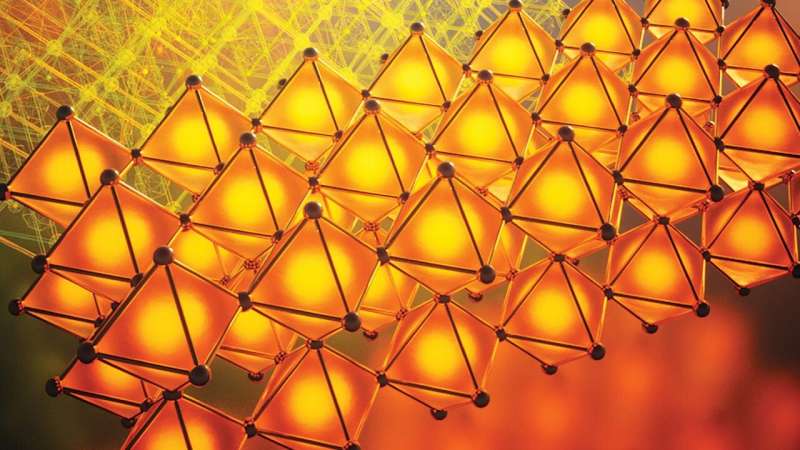
Hybrid perovskites have gained attention as a renewable energy source due to their organic-inorganic composition. However, identifying perovskites that are both efficient and can resist degradation from moisture, oxygen, light, heat, and voltage remains a major challenge.
Researchers at the University of California, Davis College of Engineering have deployed machine learning to discover high-efficiency solar cell materials by accurately predicting dynamic behaviour via fewer experiments. They have found cheaper and lighter alternatives to silicon for solar cells with similar efficiency, viable for diverse applications, including light-emitting devices.
Perovskite devices are prone to faster degradation than silicon when subjected to environmental factors like moisture, oxygen, light, heat, and voltage. Discovering those delivering high efficiency and enduring harsh conditions is vital. Perovskites’ ABX3 structure contains an organic/inorganic A group, lead/tin B, and a halide X (chlorine/iodine/fluorine). The many possible combinations make it hard to explore them with traditional trial-and-error techniques. Their evaluation under various environmental conditions also leads to a vast hyperparameter space.
High throughput experiments and machine learning
The researchers test ML algorithms to predict moisture effects on material degradation as an initial solution to the challenges. They have created a high-throughput system to measure the photoluminescence of five perovskite films under Sacramento’s summer conditions, gathering 7,000+ measurements weekly to create a reliable training set. Three machine learning algorithms were trained using the data: linear regression, neural network, and SARIMAX. The models’ predictions were compared to lab results, and SARIMAX performed best with a 90% match to observed results over 50+ hours.
Researchers believe that machine learning can identify materials and conditions preventing perovskite degradation. It can also predict the behaviour of a solar cell, with perovskite film being just a part of it.
Reference : Meghna Srivastava et al, Machine Learning Enables Prediction of Halide Perovskites’ Optical Behavior with >90% Accuracy, ACS Energy Letters (2023). DOI: 10.1021/acsenergylett.2c02555
Antonio Abate et al, Energy Spotlight, ACS Energy Letters (2023). DOI: 10.1021/acsenergylett.3c00593