ROBE Array, a breakthrough low-memory technique for deep-learning recommendation models, a form of artificial intelligence that learns to make suggestions.
Rice University computer scientists put one of the most resource-intensive forms of artificial intelligence—deep-learning recommendation models (DLRM)—within reach of small companies. DLRM system is a popular form of AI that makes relevant suggestions to its user.
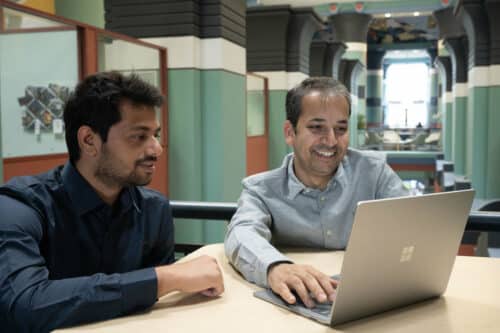
These training modules require more than a hundred terabytes of memory and supercomputer-scale processing which is only available to those companies with deep pockets. Rice’s “random offset block embedding array,” or ROBE Array can change that with its algorithmic approach for slashing the size of DLRM memory structures called embedding tables.
“ROBE Array sets a new baseline for DLRM compression,” said Anshumali Shrivastava, an associate professor of computer science at Rice. “And it brings DLRM within reach of average users who do not have access to the high-end hardware or the engineering expertise one needs to train models that are hundreds of terabytes in size.”
DLRM systems are machine learning algorithms that learn from data. One way to improve the accuracy of recommendations is to sort training data into more categories. These categorical representations are organized in memory structures called embedding tables.
“Embedding tables now account for more than 99.9% of the overall memory footprint of DLRM models,” said Aditya Desai, a Rice graduate student in Shrivastava’s research group. “This leads to a host of problems. For example, they can’t be trained in a purely parallel fashion because the model has to be broken into pieces and distributed across multiple training nodes and GPUs. And after they’re trained and in production, looking up information in embedded tables accounts for about 80% of the time required to return a suggestion to a user.”
“ROBE Array sets a new baseline for DLRM compression,” Shrivastava said. “And it brings DLRM within reach of average users who do not have access to the high-end hardware or the engineering expertise one needs to train models that are hundreds of terabytes in size.”