Researchers develop a way to make optical neural networks more precise and more prone to various hardware errors.
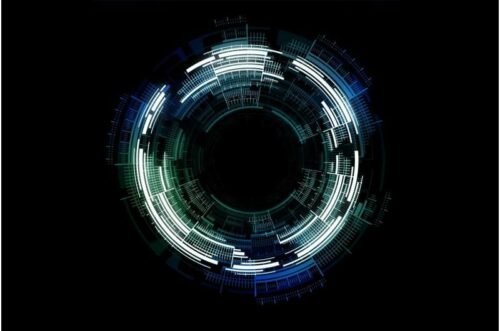
Computation models are becoming intrinsically complex, and this complexity requires to be dealt with. A solution to this problem was to replace the electrical network with an optical network. These analog devices can run many times faster while consuming far less energy. But being an analog device it becomes prone to hardware errors which can make computations less precise.
Researchers from MIT have developed a way to effectively scale an optical neural network. By adding a tiny hardware component to the optical switches that form the network’s architecture, they can reduce even the uncorrectable errors that would otherwise accumulate in the device. This could enable a super-fast, energy-efficient, analog neural network that can function with the same accuracy as a digital one. With this technique, as an optical circuit becomes larger, the amount of error in its computations actually decreases.
An optical neural network is composed of many connected components that function like reprogrammable, tunable mirrors. These tunable mirrors are called Mach-Zehnder Inferometers (MZI). Neural network data are encoded into light, which is fired into the optical neural network from a laser.
Researchers have developed a new type of MZI in which consists of an additional beam splitter to the end of the device, naming it ‘3-MZI’ because it has three beam splitters instead of two. Due to the way this additional beam splitter mixes the light, it becomes much easier for an MZI to reach the setting it needs to send all light from out through its bottom port.
The team conducted multiple simulations to test their architecture in which they found that it can eliminate much of the uncorrectable error that hampers accuracy. And as the optical neural network becomes larger, the amount of error in the device actually drops—the opposite of what happens in a device with standard MZIs. Using 3-MZIs, they could potentially create a device big enough for commercial uses with error that has been reduced by a factor of 20.
References :  “Asymptotically Fault-Tolerant Programmable Photonics”, Nature Communications (2022). dx.doi.org/10.1038/s41467-022-34308-3