A new era where machine learning meets safety in navigating complex urban terrains, promising a future where self-driving cars adapt to evolving environments without compromising safety.
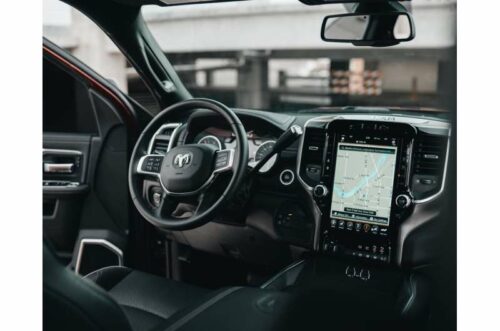
Over recent years, artificial intelligence (AI) and machine learning have been instrumental in steering self-driving cars towards advanced operational capabilities. These cars are now equipped to analyse extensive data through sensors, comprehending intricate environments and complying with traffic norms effectively. However, when transitioning from controlled environments to chaotic real-world streets, these vehicles often encounter performance disruptions, heightening the risk of accidents.
In a stride forward in the realm of autonomous vehicle technology, the NYU Tandon School of Engineering recently revealed an algorithm named Neurosymbolic Meta-Reinforcement Lookahead Learning (NUMERLA), crafted to surmount the enduring issue of unpredictability and safety in real-world scenarios. Spearheaded by Quanyan Zhu, an associate professor of electrical and computer engineering, alongside his doctoral student Haozhe Lei, the innovation is poised to revolutionise how self-driving cars adapt and function amidst the complexities of real-world traffic.
Taking a stride in bridging this gap between adaptability and safety, NUMERLA emerges as a beacon of hope. This algorithm leverages the power of real-time safety constraint updates, empowering self-driving vehicles to traverse unanticipated scenarios while firmly upholding safety protocols. Within its framework, a self-driving car, upon encountering a changing environment, fine-tunes its understanding of the current situation through collected observations. Consequently, it foresees its forthcoming performance over a predetermined period, establishing suitable safety constraints and rejuvenating its knowledge repository. This approach primarily hinges on lookahead optimisation fused with safety parameters, culminating in an empirically secure, albeit suboptimal, online control strategy. Using this algorithm, the vehicle adjusts to new circumstances expeditiously without compromising safety.
Proof of the algorithm’s efficacy was witnessed during a simulation within a digital platform mimicking urban setups, where its prowess in managing unpredictable scenarios like jaywalkers was distinctly superior to other existing algorithms.