Researchers have developed a way to make robots more intelligent with the help of self supervised learning frameworks and even teaching them psychology.
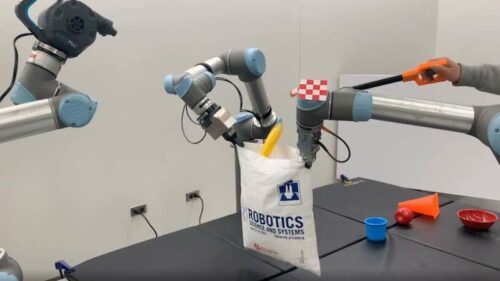
Slowly but surely, robots are improving the quality of our lives by augmenting our abilities and enhancing our safety and health. Robots can now be seen in almost every other place like a restaurant, grocery stores, malls, hospitals etc. Existing robots are becoming very efficient with simple tasks, but for performing more complex tasks they will require more development in mobility and intelligence.
Columbia Engineering and Toyota Research Institute computer scientists are looking into psychology, physics, and geometry to create algorithms so that robots can adapt to their surroundings and learn how to do things independently. Object permanence, a well-known concept in psychology that involves understanding that the existence of an object is separate from whether it is visible at any moment, is a longstanding challenge in computer vision as most applications in computer vision ignore occlusions entirely and tend to lose track of objects that become temporarily hidden from view.
To tackle this issue, researchers taught neural networks the basic physical concepts that come naturally to adults and children. Similar to how a child learns physics by watching events unfold in their surroundings, the team created a machine that watches many videos to learn physical concepts. The key idea is to train the computer to anticipate what the scene would look like in the future. By training the machine to solve this task across many examples, the machine automatically creates an internal model of how objects physically move in typical environments.
Researchers investigated how humans performed tasks intuitively to make robots more self reliable. Instead of trying to account for every possible parameter, her team developed an algorithm that allows the robot to learn from doing, making it more generalizable and lessening the need for massive amounts of training data.
Researchers modified the Iterative Residual Policy (IRP) algorithm which showed sub-inch accuracy by demonstrating it’s strong generalizing capabilities. They developed a new approach to manipulating them by using actively blown air. They armed their robot with an air pump and it was able to quickly unfold a large piece of cloth or open a plastic bag. The self-supervised learning framework they call DextAIRity learns to effectively perform a target task through a sequence of grasping or air-based blowing actions. Using visual feedback, the system uses a closed-loop formulation that continuously adjusts its blowing direction.