Machine learning (ML) technology integrated into advanced inertial sensors can improve activity tracking performance and battery life in smartphones, wearables and game controllers. Vishal Goyal, senior technical marketing manager, Analog and MEMS Group, STMicroelectronics, explains how sending lesser data to the cloud, and putting more intelligence in edges and sensors can improve overall system security and efficiency, in conversation with Deepshikha Shukla.
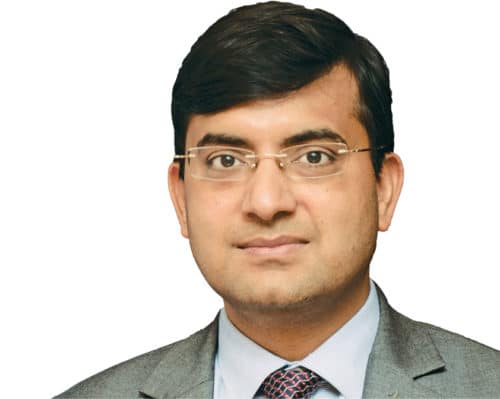
Q. What are the major industrial areas where sensors play a big role?
A. Sensors are enabling new features in all market segments. These play a major role in the automotive sector as these make the drive safer, ensure smooth navigation and a more connected vehicle. These also enable the evolution of industry towards smarter, safer, and more efficient factories and workplaces.
Sensors assist in making homes and cities smarter, connected and more aware of their surroundings to provide a better living, with higher security. Personal electronics utilise sensors like gyroscopes, accelerometers and others.
These also have a huge potential in chipsets used in communication devices like cellphones, computers and peripherals.
The basic building block to enable sensors is the semiconductor. All innovations in any industry start with innovations in the semiconductor industry.
Q. How does power consumption affect personal electronics?
A. When we move from a smartphone to an Internet of Things (IoT) device, power consumption requirement changes dramatically. We want such personal devices as smart bands to run for weeks, consume less current and be accurate. There are three macro trends for low-power personal electronics: artificial intelligence (AI), vibration and sound fusion, and shock sensing for battery monitoring. Integrating all three in sensors ensures a better user experience.
Q. What are the benefits of ML-integrated sensors?
A. When a designer is unaware of the complete scenario but develops a framework in which the algorithm can develop by itself based on data, it is called ML. That is what is being introduced in sensors, even by us.
This feat is achieved by leveraging ML tools to reduce power consumption and improve performance. ST’s sensors that have X at the end have ML code inside. Through this, we are addressing libraries for calibration, position tracking, activity-tracking and healthcare.
If you have external sensor A, you can directly interface it to ML-based sensor B instead of interfacing it to an application processor. If you interface sensor A with ML core of sensor B, sensor A will also become ML-enabled.
Q. How do low-power sensors improve overall system efficiency?
A. If a sensor consumes extremely low power but requires a microcontroller (MCU) to do a lot of tasks, then overall efficiency of the system goes down. The aim is to reduce overall system power consumption, which needs to offload the task from the MCU to the sensor.
This will lead to more reduction in power consumption in the MCU, as compared to increment in power consumption in the sensor to perform those tasks.
There are two ways for data transmission through the sensor to processor. In the first, the sensor senses and sends data continuously to the processor, so the processor is on all the time and consumes more power.
In the second, the sensor stores data continuously in its internal memory and sends it to the processor after a fixed duration. Overall time that the MCU is on reduces dramatically, which helps achieve overall much lower power consumption.
In edge computing, instead of taking decisions at the cloud, decisions are taken at sensor node itself. Data sent to the cloud is based on those decisions. With this, amount of data going to the cloud reduces, network bandwidth reduces and, hence, overall cost of operation and power consumption reduces. Overall power consumption at the MCU reduces to zero, with intelligence transferred to the sensor.
Q. How do decision trees in ML help make decisions for sensors?
A. ML core works in conjunction with the sensor’s integrated finite-state machine logic to handle motion pattern recognition or vibration detection. Those creating activity-tracking products can train the core for decision-tree-based classification using Weka (ST’s patented technology)—an open-source PC-based application—to generate settings and limits from sample data such as acceleration, speed and magnetic angle that characterise the types of movement to be detected.
Q. How do ML-based sensors compute and transfer data faster than normal sensors?
A. ML-based sensors have more internal memory than conventional ones, and a state-of-the-art high-speed I3C (combination of SPI bus and I2C bus) digital interface, allowing longer periods between interactions with the main controller, and shorter connection times for extra energy savings.
For example, ST’s LSM6DSOX iNEMO sensor contains a ML core to classify motion data based on known patterns. We are targeting this sensor for smartphones, smart bands, wearables, game controllers and IoT devices. It has a dedicated OIS core for an auxiliary interface, which can connect up to four external sensors. It has very high accuracy, finite-state machine, 3D MEMS accelerometer, 3D MEMS gyroscope and can store up to 9kB into the sensor itself.