Researchers at The Ohio State University have developed a new approach to predict the behavior of spatiotemporal chaotic systems that are particularly complex for scientists to forecast
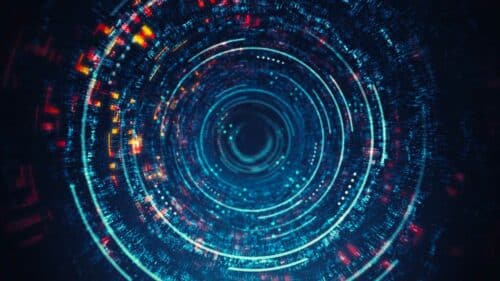
Traditional machine learning algorithm used for forecasting the behavior of an atmospheric weather model is expensive and needs a large amount of training data for predictions. Hence, researchers implemented a new and highly efficient algorithm that when integrated with next-generation reservoir computing, could learn spatiotemporal chaotic systems in less time compared to other machine learning algorithms.
“Modern machine learning algorithms are especially well-suited for predicting dynamical systems by learning their underlying physical rules using historical data,” said De Sa Barbosa. “Once you have enough data and computational power, you can make predictions with machine learning models about any real-world complex system.” Such systems can include any physical process, from the bob of a clock’s pendulum to disruptions in power grids.
The Ohio State team’s algorithm is more precise and needs 400 to 1,250 times less training data to make better predictions than its counterpart. Their method is also less computationally expensive. previously solving complex computing problems required a supercomputer while they used a laptop running Windows 10 to make predictions in about a fraction of a second which is about 240,000 times faster than traditional machine learning algorithms. Even heart cells display chaotic spatial patterns when they oscillate at an abnormally higher frequency than a normal heartbeat. That means this research could one day be used to provide better insight into controlling and interpreting heart disease, as well as a bevy of other “real-world” problems. This new machine learning algorithm only needs almost 400 historical training data points for the atmospheric weather example while previously it needed almost 500,000, and still achieves the same or better accuracy.
“This is very exciting, as we believe it’s a substantial advance in terms of data processing efficiency and prediction accuracy in the field of machine learning,” said Wendson De Sa Barbosa, lead author and a postdoctoral researcher in physics at Ohio State. He said that learning to predict these extremely chaotic systems is a “physics grand challenge,” and understanding them could pave the way to new scientific discoveries and breakthroughs.
“We live in a world that we still know so little about, so it’s important to recognize these high-dynamical systems and learn how to more efficiently predict them.”
Click here for the Published Research Paper