The adoption of artificial intelligence (AI) and machine learning (ML) in different sectors has transformed the conventional approach for different applications. Although the terms AI and ML are used interchangeably, the former aims at the success of a task, whereas the latter ensures accuracy.
From marketing and retail to healthcare and finance, adoption of artificial intelligence (AI) and machine learning (ML) in these sectors is drastically transforming the conventional approach for different applications. AI makes it possible for systems to sense, comprehend, act, and learn for performing complex tasks such as decision making that earlier required human intelligence. Unlike the regular programming, where action needs to be defined for every situation, AI in conjunction with ML algorithms can process large data sets, be trained to choose how to respond, and learn from every problem it encounters to produce more accurate results.
This has not only impacted how we use data but also how we design and fabricate hardware chips or integrated circuits (ICs) for enabling next-generation devices, thereby opening new opportunities. The growth of AI shifts the core of innovation from the software back to hardware. For better performance, for example, AI needs more memory as compared to traditional requirements to process and transfer large data sets. Consider the case of virtual assistants that are increasingly being used in homes. Without reliable hardware for such functionalities as those associated with memory and logic, these cannot work properly.
According to Accenture Semiconductor Technology Vision 2019, from the annual report by the Accenture Labs and Accenture Research, the semiconductor industry is highly optimistic about the potential of AI in their work in the coming years when compared to that in technologies like extended reality, distributed ledgers, and quantum computing. Three-quarters (77 per cent) of semiconductor executives surveyed for the report claimed that they either had already adopted AI within their business or were piloting the technology.
The concept of AI and ML
Although the terms AI and ML are used interchangeably, the former aims at the success of a task, whereas the latter ensures accuracy. So, solving a complex problem is done through AI training, but maximising efficiency by learning from the data and already performed tasks is the concept of ML. ML relies on large datasets to find common patterns and makes predictions based on probability.
Applied AI is more commonly seen in systems that are designed for financial market predictions and autonomous vehicle control. Generalised AIs to handle different general situations is where ML, usually considered the subset of AI, comes into play. In what is called supervised learning ML algorithm, the relationship model between input data and target output is established to make predictions, whereas unsupervised learning does not involve categorising data for training. In situations like competing with humans in complex computer games where information needs to be gained from the environment continuously, reinforcement learning is implemented.
Sub-branches of ML like deep neural networks have already been applied to fields, including speech recognition, social network filtering, computer vision, natural language processing, and so on. These technologies take and examine thousands of users’ data for precision and accuracy applications like face recognition. This is contributing to the rapid development of innovations that are considered magical right now, but with hardware advancements, its place might be taken by much more advanced innovations in the coming decades.
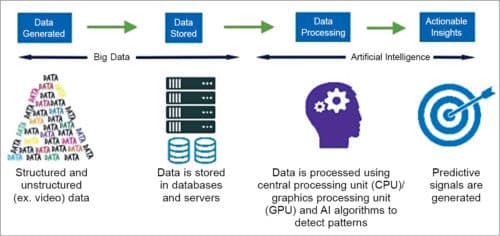
How AI and ML applications are redefining the traditional systems
AI and ML could grow to the current extent due to advancements in not only algorithms but also in storage capabilities, computation capacity, networking, and the like, which made it possible to make advanced devices accessible to the masses at an economical cost. Traditionally, logic was usually hard-wired in the design of electronic systems. But in the light of high manufacturing costs presently and the growing complexity of chip development, AI-driven processor architectures are redefining traditional processor architectures to suit new demands.
Computation is mainly done on the central processing unit (CPU), the brain of the computer. With the emergence of computationally demanding applications that apply AI and ML algorithms, additional processing choices through combinations of graphics processing units (GPUs), microprocessors (MPUs), microcontrollers (MCUs), field-programmable gate arrays (FPGAs), and digital signal processors (DSPs) are coming up to meet the optimum feature requirements. The options, considered distinct categories, are gradually becoming heterogeneous processing solutions like system-on-chips (SoCs) and custom-designed application-specific integrated circuits (ASICs).
Unlike traditional storage methods to store parameters while tuning a neural network model during training and inference, high-bandwidth RAM is required not only at data centres but also for edge computing. The increased amount of volatile memory for proper functioning causes an excessive rise in power consumption levels. This is driving the need for evolving memory interfaces to ensure that the tasks get executed at high speeds. Although new processor architectures are helping reduce the load, other mechanisms like new memory interfaces and processing into memory itself are also being researched upon and implemented.
UK-based startup Graphcore’s IPU is a novel processor with high on-chip memory capacity specifically designed for dealing with complex data structures in machine intelligence models. Outside memory sources take much more time to return a result than on-chip ones. According to a report from IHS Markit, worldwide revenue from memory devices in AI applications will increase to 60.4 billion dollars in 2025 from 20.6 billion dollars in 2019, while the processor segment will grow from 22.2 billion dollars in 2019 to 68.5 billion dollars in 2025.
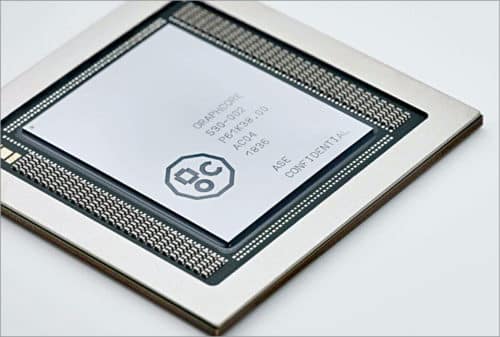
Thus, semiconductors provide the necessary processing and memory capabilities required for all AI applications. Improving network speed is also important for working with multiple servers simultaneously and developing accurate AI models. Measures such as high-speed interconnections and routing switches are being examined for load balancing.
The answer to improving chips for AI applications lies with the technology itself. AI and ML are being leveraged to improve performance, and as design teams become more experienced in this field, they will enhance how chips are developed, manufactured, and tweaked for updates. Complicated issues related to the implementation, signoff, and verification of chips that cannot be solved and optimised with traditional methods can be solved with AI and ML.
Using ML-based predictive models inside the existing EDA tools, US-based company Synopsys claims to have achieved results like a five times faster PrimeTime power recovery and a hundred times faster high sigma simulation in HSPICE. All this requires a focus on R&D and accurate end-to-end solutions, creating opportunities for possibly entirely new markets with value-creation in different segments of semiconductor companies.
Overcoming the challenges
The main focus is on data and its usage. This requires more than just a new architecture for the processor. Different chip architectures work for different purposes, and factors like the size and value of the training data can render AI useless for some applications.
AI makes it possible to process data as patterns instead of individual bits and works best when memory operations are to be done in the form of a matrix, thereby increasing the amount of data being processed and stored and hence the efficiency of the software. For instance, spiking neural networks can reduce the flow of data as the data is fed in the form of spikes. Also, even if there is a lot of data, the amount of useful data to train a predictive model can be reduced. But the issues still exist. Like in chip design, the training of ML models happens in different environments independently at different levels.
There needs to be a standardised approach to the application of AI. For efficient utilisation of AI, chips designed for AI, as well as those modified to suit AI requirements, need to be considered. If there is a problem in the system, there is a need for tools and methodologies to quickly solve it. The design process is still highly manual despite the growing adoption of design automation tools. Tuning the inputs is a time-consuming yet highly inefficient process. Even just one small step in a design implementation can be an entirely new problem itself.
There has been widespread misuse by companies claiming to use AI and ML to gain the advantage of the trend and increase sales and revenue. Although the cost of compression and decompression is not so high, the cost of on-chip memory is not so cheap. AI chips also tend to be very large.
To build such systems that store and process data, the collaboration of experts on different teams is necessary. Major chipmakers, IP vendors, and other electronics companies will be adopting some form of AI to increase efficiency across their operations. The availability of cloud compute services at a reduced cost of computing can help in boosting evolution. Technology advancements will force semiconductor companies to empower and reskill their workforces, enabling the next generation of devices in the marketplace.