Having a cool technology like artificial intelligence (AI) at our disposal was never meant to be a panacea, and we should stop being carried away with it. If AI is such a great thing, why should we focus and limit its applications in solving problems? Would it not be better to pre-empt problems and avoid them altogether?
Poorly designed artificial intelligence (AI) is nothing short of banana!
These days we are so gung-ho about new technologies—mainly AI—that we have almost stopped demanding good-quality solutions. We are so fond of this newness that we are ignoring flaws new technologies are carrying over. This, in turn, is encouraging sub-par solutions day-by-day, and we are sitting on a pile of junk, which is growing faster than ever. The disturbing part of that is, those who are making sub-par solutions, still, make us believe that machines will be wiser soon. When and how, I wonder! More importantly, why should we tolerate poorly-designed solutions until then?
Having a cool technology at our disposal was never meant to be a panacea, and we should stop being carried away with it. If AI is such a great thing—I know it is, but not in its current form—why should we focus and limit its applications in solving problems? I would rather pre-empt problems and avoid them altogether; wouldn’t that be a wise thing to do? How many technologies stop problems from becoming problems today?
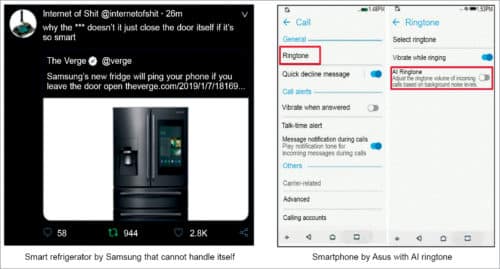
I think we should avoid rushing towards and following trends and hypes blindly. Rather, we must become thoughtful and maintain our sanity in deciding where, when and why we use emerging technologies, AI included.
When I mention junk, I am referring to some of the sub-par and trivial solutions that add low to no value. I am sure that the community would tend to disavow them, but be known that these have already made their way to the crowd anyway.
The figure above shows only a couple of examples, which are merely the tip of an iceberg of AI junk out there. Things like AI ringtones and other similar (so-called smart) things really need to stop polluting the real technology market.
Why we need to challenge this kind of stuff
Well, first, I am not against emerging technologies at all; rather, I am a strong proponent. However, what I do not quite appreciate is quantity over quality. I think it often promotes false economics, and here is why.
- Simply pushing for more quantity (of tech solutions) without paying attention to quality (of solutions) adds a lot of garbage out in the field.
- This garbage, when out there in the market, creates disillusionment and, in turn, adds to the resistance in healthy adoption.
- This approach (quantity versus quality) also encourages bad practices by creators of AI at customers’ expense, where sub-par solutions are pushed down their throat by leveraging their ignorance and an urge to give in to FOMO (fear of missing out).
Stuff like this happens during the hype cycle for any new and upcoming trend or technology. If this is happening with AI, there is nothing new, per se.
The problem though is that flaws in sub-par technologies do not surface until it is too late. In many cases, the damage is already done and is irreversible. Unlike many other technologies, where things simply work or do not work, there is a significant grey area that can change its shades over time. Moreover, if we are not clear in which direction that would be, we end up creating junk.
We not only need to challenge the quality of every solution but also improvise our approach towards emerging technologies in general.
How we can encourage a better approach
There is no golden rule or checklist as such for a better approach. Nonetheless, a few things I can suggest are:
Seeking transparency in solution
You would notice that people who make something often replicate their inner self in the output. A casual approach or thinking would encourage a casual output, and so would the meticulous thinking result in better output. Programmers who have clarity of mind and cleanliness in behaviour often create a clean and flawless program.
We must make people responsible and accountable for their output. In case of AI, if it does a bad job, ask creators to explain and seek transparency. Hiding behind deep learning algorithms and saying, “AI did it!” will not cut it.
People will often tell you that knowing inner mechanics is not important for users, only the output is. We must challenge this argument. For many other technologies, such as a car or microwave oven, this argument might hold true, but for AI, it does not!
For each action automation or AI takes, creators should be able to explain why their technology did that.
Seeking quality and accountability of outcomes
People may (most likely) rely on technology and automation for their business-critical or life-critical functions. Bringing in people who would take accountability of outcomes seriously is often a better decision. Unless the application is for fancy or sheer playfulness, we need to put a responsible adult in charge.
Machine learning is essentially teaching a computer to label things with examples rather than with programmed instructions. In addition, AI that is based on this learning can do wrong things only in two circumstances. First, if the fundamental logic of decision making programmed in it (by humans) is flawed, that is, bad programming; or the dataset it was trained on is incorrectly labelled (by humans), that is, of bad data quality. In either case, the machine will do the wrong thing or do it wrong!
Here, creators cannot just wash their hands off and say, they do not know what went wrong when something goes awry. Humans need to take responsibility on embedding those wrong fundamentals and take accountability of outcomes.
The world, even today, looks at parents if their child does something wrong, questioning their upbringing methods and practices. Then why cannot we look at the creators of AI solutions the same way?
We should start assigning liability to creators of AI, much as we do now with guns, and make quality acceptance parameters stricter.
Being careful with MVP people
No doubt that a minimum viable product, aka MVP, is key to developing a successful product. However, treating MVP as MVP is very important.
The problem starts when someone sells you his or her work, which is still in progress and has not achieved an acceptable working level yet. The solution not working would be less of a problem than it is working against your goals.
The issue with many AI or automation solutions is that sometimes these do not break evidently. These create a leakage of some sort, which is not quite bad but not quite right either. This sort of grey output piles over a period before one can realise that they own a heap of junk. Getting rid of that junk or fixing the completely-flawed system could become a nightmare.
MVP is good and important, but we should deal with solutions that are not ready for full live deployment with utmost care and skepticism.
Auditing the technology thoroughly
Contemporary solutions are more than simply packaged software these days. These are complex pieces of intertwined technologies. Evidently, older ways of ensuring that these solutions are working as expected are not suitable anymore. Quality-assurance methods such as unit testing, regression testing and so on are not enough to qualify emerging technological solutions. We rather need to take a systematic approach, and test the technology for real-life scenarios, possible and impossible (scenarios) both at the same level.
Thoroughly audit the system for what it has learnt, how and from whom, and then verify how that can affect you in the future. Do not just take the creators’ word for it.
Most importantly, pull the plug if needed. It is too costly to keep going with bad-quality automation and AI than to just shut it.
Preferring pre-emption over a fix
There are several solutions (for one problem) in the market, even now, at a nascent stage. Not all solutions can be a good match. Sometimes, their offerings are slightly different. Some solutions can provide a fix to the problem as an after the fact (for example, automated fixing of a broken machine), while some would provide an early indication (for example, anomaly detection in machine performance). Some will help in improving problem-fixing performance, while some will help in identifying problems early on.
Focus and prefer pre-emptive systems than fixers. Both are important, but if you must, prevention is always better than cure!
Avoiding ignorance from the top
People often leverage your ignorance to sell you something. Getting educated on emerging technologies is quite important to avoid these miss-sells. Starting from the top is even more important. Executives should understand better as to what they are getting into. They need to invest some time in acquiring this education to be able to ask the right questions at the right time/steps. Being more involved is necessary; simply learning some tech-lingo is not going to be enough. If you are not involved, nothing else can fix it, ever!
Adding feedback loop and sincerely learning from it
Unfortunately, there is a general absence of experience-based knowledge in the developer community, so we cannot rely solely on data and algorithms to know the right problems to solve. This is a great issue these days, and neutral business perspective is often required to see things through the objectivity lens.
My mention here of feedback loop does not relate to machine learning or AI system as such; it refers to the development and use of value chain. When users provide feedback to creators and creators learn from it to adjust their creation, feedback serves better. If creators consume feedback but never consider or act on it, it remains an open loop system. The open loop system is not a stable one.
Creators and users should ensure that an effective feedback loop is established and everyone in that loop is sincerely learning from it.
As more and more technology is democratised, the ability to create junk (knowingly or unknowingly) would increase manifold. While some will use wisdom in creating sensible solutions that are of great quality and useful, many others would do just the opposite. We must brace ourselves; first to stop this junk from coming in, and second to tackle it, if it comes through!
Anand Tamboli is a serial entrepreneur, senior emerging technologies leader and advisor, and author of Build Your Own IoT Platform book